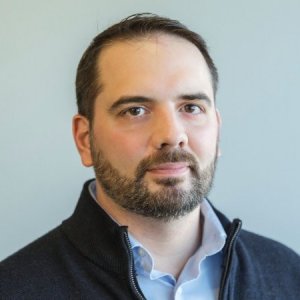
Dean Krusienski, Ph.D.
Professor and Graduate Program Director, Department of Biomedical Engineering | B.S., M.S., Ph.D., The Pennsylvania State University
- Richmond VA UNITED STATES
Focusing on neural signal processing and analysis for the development of brain-computer interfaces and neuroprosthetic devices.
Social
Biography
Areas of Expertise
Education
The Pennsylvania State University
Doctor of Philosophy
Electrical Engineering
2004
The Pennsylvania State University
Master of Science
Electrical Engineering
2001
The Pennsylvania State University
Bachelor of Science
Electrical Engineering
1999
minor in Biomedical Engineering
Research Focus
Brain-Computer Interfaces
Focusing on neural signal processing and analysis for the development of brain-computer interfaces and neuroprosthetic devices.
Research Grants
EAGER: EEG-based Cognitive-state Decoding for Interactive Virtual Reality
NSF
2019-10-01
The increasing availability of affordable, high-performance virtual reality (VR) headsets creates great potential for applications including education, training, and therapy. In many applications, being able to sense a user's mental state could provide key benefits. For instance, VR environments could use brain signals such as the electroencephalogram (EEG) to infer aspects of the user's mental workload or emotional state; this, in turn, could be used to change the difficulty of a training task to make it better-suited to each user's unique experience. Using such EEG feedback could be valuable not just for training, but in improving people's performance in real applications including aviation, healthcare, defense, and driving. This project's goal is to develop methods and algorithms for integrating EEG sensors into current VR headsets, which provide a logical and unobtrusive framework for mounting these sensors. However, there are important challenges to overcome. For instance, EEG sensors in labs are typically used with a conducting gel, but for VR headsets these sensors will need to work reliably in "dry" conditions without the gel. Further, in lab settings, motion isn't an issue, but algorithms for processing the EEG data will need to account for people's head and body motion when they are using headsets.
To address these challenges, the project team will build on recent advances in dry EEG electrode technologies and motion artifact suppression algorithms, focusing on supporting passive monitoring and cognitive state feedback. Such passive feedback is likely to be more usable in virtual environments than active EEG feedback, both because people will be using other methods to interact with the environment directly and because passive EEG sensing is more robust to slower response times and decoding errors than active control. Prior studies have demonstrated the potential of EEG for cognitive-state decoding in controlled laboratory scenarios, but practical EEG integration for closed-loop neurofeedback in interactive VR environments requires addressing three critical next questions: (1) can more-practical and convenient EEG dry sensors achieve comparable results to wet sensors?, (2) can passive EEG cognitive-state decoding be made robust to movement-related artifacts?, and (3) can these decoding schemes be generalized across a variety of cognitive tasks and to closed-loop paradigms? To address these questions, classical cognitive tasks and more-complex sim
US-German Data Sharing Proposal: CRCNS Data Sharing: REvealing SPONtaneous Speech Processes in Electrocorticography (RESPONSE)
NSF
2016-08-01
The uniquely human capability to produce speech enables swift communication of abstract and substantive information. Currently, nearly two million people in the United States, and far more worldwide, suffer from significant speech production deficits as a result of severe neuromuscular impairments due to injury or disease. In extreme cases, individuals may be unable to speak at all. These individuals would greatly benefit from a device that could alleviate speech deficits and enable them to communicate more naturally and effectively. This project will explore aspects of decoding a user's intended speech directly from the electrical activity of the brain and converting it to synthesized speech that could be played through a loudspeaker in real-time to emulate natural speaking from thought. In particular, this project will uniquely focus on decoding continuous, spontaneous speech processes to achieve more natural and practical communication device for the severely disabled.
The complex dynamics of brain activity and the fundamental processing units of continuous speech production and perception are largely unknown, and such dynamics make it challenging to investigate these speech processes with traditional neuroimaging techniques. Electrocorticography (ECoG) measures electrical activity directly from the brain surface and covers an area large enough to provide insights about widespread networks for speech production and understanding, while simultaneously providing localized information for decoding nuanced aspects of the underlying speech processes. Thus, ECoG is instrumental and unparalleled for investigating the detailed spatiotemporal dynamics of speech. The research team's prior work has shown for the first time the detailed spatiotemporal progression of brain activity during prompted continuous speech, and that the team's Brain-to-text system can model phonemes and decode words. However, in pursuit of the ultimate objective of developing a natural speech neuroprosthetic for the severely disabled, research must move beyond studying prompted and isolated aspects of speech. This project will extend the research team's prior experiments to investigate the neural processes of spontaneous and imagined speech production. In conjunction with in-depth analysis of the recorded neural signals, the researchers will apply customized ECoG-based automatic speech recognition (ASR) techniques to facilitate the analysis of the large amount of phones occurring in contin
EAGER: Investigating the Neural Correlates of Musical Rhythms from Intracranial Recordings
NSF
2014-09-01
The project will develop an offline and then a real-time brain computer interface to detect rhythms that are imagined in people's heads, and translate these rhythms into actual sound. The project builds upon research breakthroughs in electrocorticographic (ECoG) recording technology to convert music that is imagined into synthesized sound. The project researchers will recruit from a specialized group of people for this project, specifically patients with intractable epilepsy who are currently undergoing clinical evaluation of their condition at the Mayo Clinic in Jacksonville, Florida, and are thus uniquely prepared to use brain-computer interfaces based on ECoG recording techniques. This is a highly multidisciplinary project that will make progress towards developing a "brain music synthesizer" which could have a significant impact in the neuroscience and musical domains, and lead to creative outlets and alternative communication devices and thus life improvements for people with severe disabilities.
Most brain-computer interfaces (BCIs) use surface-recorded electrophysiological measurements such as surface-recorded electroencephalogram (EEG). However, while some useful signals can be extracted from such surface techniques, it is nearly impossible to accurately decode from such signals the intricate brain activity involved in activities such as language with the detail needed to achieve a natural, transparent translation of thought to device control. On the contrary, intracranial electrodes such as ECoG are closer to the source of the desired brain activity, and can produce signals that, compared to surface techniques, have superior spatial and spectral characteristics and signal-to-noise ratios. Research has already shown that intracranial signals can provide superior decoding capabilities for motor and language signals, and for BCI control. Because complex language and auditory signals (both perceived and imagined) have been decoded using intracranial activity, it is conceivable to decode perceived and imagined musical content from intracranial signals. This project will attempt to similarly use ECoG to decode perceived and imagined musical content from intracranial signals as has been done for language and auditory signals.
CHS: Small: A Hybrid Brain-Computer Interface for Behaviorally Non-Responsive Patients
NSF
2014-08-01
Brain-computer interfaces (BCIs) have been explored for several years in an effort to provide communication for "locked in" users who have the desire and mental capacity to communicate but are unable to speak, type, or use conventional assistive technologies due to severe motor disabilities, and a lot of work has gone into making this initially crude technology more practical, usable, accurate, and flexible (e.g., by improving speed of performance and providing virtual reality feedback and/or advanced device control). In this project the PI and his team turn their attention to a group with even greater need: patients who have been misdiagnosed as vegetative or minimally conscious, and without the mental ability to form messages or respond to questions. These individuals are not only unable to move but also unable to see, and are at risk of being euthanized based on the mistaken assumption that they are effectively "brain dead" whereas it has been shown by European colleagues, using methods and equipment comparable to American hospitals, that 17-42% of such patients were in fact able to use a BCI to respond to questions. The PI worries that severely injured veterans and others might sometimes be misdiagnosed, potentially able to communicate with friends and loved ones if only some technology could more effectively assess their brain activity. The PI's goal in this project is to extend current BCI technologies to focus on assessing consciousness in this vulnerable patient population and provide, where possible, the ability to communicate.
The PI's approach is to adapt and extend conventional BCI protocols and feedback environments to work with people who cannot see and must instead rely on other modalities of stimulation. The work will involve three thrusts. First, the PI and his team will improve methods to identify brain response based only on tactile and auditory stimuli, by determining the best tactile stimulation frequency for each subject. Second, they will use "hybrid" BCIs combining P300s and steady state somatosensory evoked potentials (SSSEPs) to elicit two different kinds of EEG signals that could improve accuracy. Finally, they will develop a new six choice BCI system tailored for these users; at present the best BCIs for these patient groups allow just two or three choices, whereas a six choice system could lead to faster communication and broader control options. Across all three of these thrusts, the team will also explore signal processing m
Classifier Development forSteady-State Visually Evoked Potential Data
NIA/NASA
2015-09-01
Classifier Development forSteady-State Visually Evoked Potential Data
MRI Consortium: Acquisition of an Integrated System of Instruments for Multichannel Biopotential Recording of In-vitro and In-vivo Experiments
NSF
2013-09-01
An award is made to the Norfolk State University (NSU) to build an integrative research platform for neural recording and analysis and provide the resources for students, faculty and associated collaborators for their research activities from electronic and optical engineering, biology, computer science, nanotechnology, immunology, and neuroscience fields. The addition of this system to the existing research infrastructures will directly benefit and upgrade investigator's research programs at NSU, Eastern Virginia Medical School and Old Dominion University, as well as the collaborative network capabilities in the Hampton Roads area. Applying the multichannel biopotential recording system, neural and cardiac potentials, and neurochemicals such as neurotransmitters can be measured in-vivo and in-vitro, and analyzed by embedded software. The instrument will be applied for the study of neural mechanisms underlying the interactions under various behavioral, stimuli, and disease conditions. In addition to the biopotential recording, the instrumentation will allow in-vivo assessment of neural sensing devices to be developed by engineering research. Furthermore, in-vivo and in-vitro real-time measurement and monitoring of sensing signal can be applied for the development of novel therapeutic treatment strategies of neurological disorders and degeneration.
The outcomes of this synergetic research will be positively transferred to achieve their STEM educational and outreach goals, especially focused for under-represented minority (URM) students in the project?s leading institution, NSU, one of the largest Historically Black Colleges and Universities in the U.S. This project will specifically place a high priority on training of URM students in Hampton Roads region and extend their efforts to URM students nationwide through an aggressive set of research, training and outreach activities utilizing current outreach programs on each campus. The outcomes of this project will be incorporated in their Bioengineering Minor program curriculum. Furthermore, they will also integrate this infrastructure with activity of the newly created Hampton Roads Neuroscience Network.
HCC: Medium: RUI: Control of a Robotic Manipulator via a Brain-Computer Interface
NSF
2009-07-15
A brain-computer interface (BCI) is a system that allows users, especially individuals with severe neuromuscular disorders, to communicate and control devices using their brain waves. There are over two million people in the United States afflicted by such disorders, many of whom could greatly benefit from assistive devices controlled by a BCI. Over the past two years, it has been demonstrated that a non-invasive, scalp-recorded electroencephalography (EEG) based BCI paradigm can be used by a disabled individual for long-term, reliable control of a personal computer. This BCI paradigm allows users to select from a set of symbols presented in a flashing visual matrix by classifying the resulting evoked brain responses. One of the goals of this project is to establish that the same BCI paradigm and techniques used for the aforementioned demonstration can be straightforwardly implemented to generate high-level commands for controlling a robotic manipulator in three dimensions according to user intent, and that such a BCI can provide superior dimensional control over alternative BCI techniques currently available, as well as a wider variety of practical functions for performing everyday tasks.
Electrocorticography (ECoG), electrical activity recorded directly from the surface of the brain, has been demonstrated in recent preliminary work to be another potentially viable control for a BCI. ECoG has been shown to have superior signal-to-noise ratio, and spatial and spectral characteristics, compared to EEG. But the EEG signals used at present to operate BCIs have not been characterized in ECoG. The PI believes ECoG signals can be used to improve the speed and accuracy of BCI applications, including for example control of a robotic manipulator. Thus, additional goals of this project are to characterize evoked responses obtained from ECoG, to use them as control signals to operate a simulated robotic manipulator, and to assess the level of control (speed and accuracy) between the two recording modalities and compare the results to competitive BCI techniques. Because this is a collaborative effort with the Departments of Neurology and Neurosurgery at the Mayo Clinic in Jacksonville, the PI team will have access to a pool of ECoG grid patients from which to recruit participants for this study.
Broader Impacts: This research will make a number of contributions in the emerging field of BCI and thus will serve as a step toward providing severely disabled individuals
Courses
EGRB 603. Biomedical Signal Processing
Explores theory and application of discrete-time signal processing techniques in biomedical data processing. Includes discrete-time signals and systems, the Discrete/Fast Fourier Transforms (DFT/FFT), digital filter design and implementation, and an introduction into processing of discrete-time random signals.
EGRB 601. Numerical Methods and Modeling in Biomedical Engineering
The goal of this course is to develop an enhanced proficiency in the use of computational methods and modeling, to solve realistic numerical problems in advanced biomedical engineering courses and research, as well careers. The course will discuss and students will develop advanced technical skills in the context of numerical data analysis and modeling applications in biology and medicine. An important component of this course is developing problem-solving skills and an understanding of the strengths and weaknesses of different numerical approaches applied in biomedical engineering applications.
EGRB 308. Biomedical Signal Processing
Explores the basic theory and application of digital signal processing techniques related to the acquisition and processing of biomedical and physiological signals including signal modeling, AD/DA, Fourier transform, Z transform, digital filter design, continuous and discrete systems.
Selected Articles
Prefrontal High Gamma in ECoG Tags Periodicity of Musical Rhythms in Perception and Imagination
eNeuroSA Herff, C Herff, AJ Milne, GD Johnson, JJ Shih and DJ Krusienski
2020-06-25
Rhythmic auditory stimuli are known to elicit matching activity patterns in neural populations. Furthermore, recent research has established the particular importance of high-gamma brain activity in auditory processing by showing its involvement in auditory phrase segmentation and envelope tracking. Here, we use electrocorticographic (ECoG) recordings from eight human listeners to see whether periodicities in high-gamma activity track the periodicities in the envelope of musical rhythms during rhythm perception and imagination. Rhythm imagination was elicited by instructing participants to imagine the rhythm to continue during pauses of several repetitions. To identify electrodes whose periodicities in high-gamma activity track the periodicities in the musical rhythms, we compute the correlation between the autocorrelations (ACCs) of both the musical rhythms and the neural signals. A condition in which participants listened to white noise was used to establish a baseline. High-gamma autocorrelations in auditory areas in the superior temporal gyrus and in frontal areas on both hemispheres significantly matched the autocorrelations of the musical rhythms. Overall, numerous significant electrodes are observed on the right hemisphere. Of particular interest is a large cluster of electrodes in the right prefrontal cortex that is active during both rhythm perception and imagination. This indicates conscious processing of the rhythms’ structure as opposed to mere auditory phenomena. The autocorrelation approach clearly highlights that high-gamma activity measured from cortical electrodes tracks both attended and imagined rhythms.
The Potential of Stereotactic-EEG for Brain-Computer Interfaces: Current Progress and Future Directions
Frontiers in Neuroscience, (Neuroprosthetics)C Herff, DJ Krusienski, P Kubben
2020-02-27
Stereotactic electroencephalogaphy (sEEG) utilizes localized, penetrating depth electrodes to measure electrophysiological brain activity. It is most commonly used in the identification of epileptogenic zones in cases of refractory epilepsy. The implanted electrodes generally provide a sparse sampling of a unique set of brain regions including deeper brain structures such as hippocampus, amygdala and insula that cannot be captured by superficial measurement modalities such as electrocorticography (ECoG). Despite the overlapping clinical application and recent progress in decoding of ECoG for Brain-Computer Interfaces (BCIs), sEEG has thus far received comparatively little attention for BCI decoding. Additionally, the success of the related deep-brain stimulation (DBS) implants bodes well for the potential for chronic sEEG applications. This article provides an overview of sEEG technology, BCI-related research, and prospective future directions of sEEG for long-term BCI applications.
Generating Natural, Intelligible Speech From Brain Activity in Motor, Premotor, and Inferior Frontal Cortices
Front. Neurosci.C Herff, L Diener, M Angrick, E Mugler, M Tate, M Goldrick, DJ Krusienski, M Slutzky, T Schultz
2019-11-22
Neural interfaces that directly produce intelligible speech from brain activity would allow people with severe impairment from neurological disorders to communicate more naturally. Here, we record neural population activity in motor, premotor and inferior frontal cortices during speech production using electrocorticography (ECoG) and show that ECoG signals alone can be used to generate intelligible speech output that can preserve conversational cues. To produce speech directly from neural data, we adapted a method from the field of speech synthesis called unit selection, in which units of speech are concatenated to form audible output. In our approach, which we call Brain-To-Speech, we chose subsequent units of speech based on the measured ECoG activity to generate audio waveforms directly from the neural recordings. Brain-To-Speech employed the user's own voice to generate speech that sounded very natural and included features such as prosody and accentuation. By investigating the brain areas involved in speech production separately, we found that speech motor cortex provided more information for the reconstruction process than the other cortical areas.
Estimating Cognitive Workload in an Interactive Virtual Reality Environment Using EEG
Front. Hum. Neurosci.C Tremmel, C Herff, T Sato, K Rechowicz,Y Yamani, DJ Krusienski. Estimating Cognitive Workload in an Interactive VR Environment using EEG
2019-11-14
With the recent surge of affordable, high-performance virtual reality (VR) headsets, there is unlimited potential for applications ranging from education, to training, to entertainment, to fitness and beyond. As these interfaces continue to evolve, passive user-state monitoring can play a key role in expanding the immersive VR experience, and tracking activity for user well-being. By recording physiological signals such as the electroencephalogram (EEG) during use of a VR device, the user's interactions in the virtual environment could be adapted in real-time based on the user's cognitive state. Current VR headsets provide a logical, convenient, and unobtrusive framework for mounting EEG sensors. The present study evaluates the feasibility of passively monitoring cognitive workload via EEG while performing a classical n-back task in an interactive VR environment. Data were collected from 15 participants and the spatio-spectral EEG features were analyzed with respect to task performance. The results indicate that scalp measurements of electrical activity can effectively discriminate three workload levels, even after suppression of a co-varying high-frequency activity.
Speech synthesis from ECoG using densely connected 3D convolutional neural networks
Journal of Neural EngineeringM Angrick, C Herff, E Mugler, M Tate, M Slutzky, DJ Krusienski, T Schultz
2019-04-16
Objective. Direct synthesis of speech from neural signals could provide a fast and natural way of communication to people with neurological diseases. Invasively-measured brain activity (electrocorticography; ECoG) supplies the necessary temporal and spatial resolution to decode fast and complex processes such as speech production. A number of impressive advances in speech decoding using neural signals have been achieved in recent years, but the complex dynamics are still not fully understood. However, it is unlikely that simple linear models can capture the relation between neural activity and continuous spoken speech. Approach. Here we show that deep neural networks can be used to map ECoG from speech production areas onto an intermediate representation of speech (logMel spectrogram). The proposed method uses a densely connected convolutional neural network topology which is well-suited to work with the small amount of data available from each participant. Main results. In a study with six participants, we achieved correlations up to r = 0.69 between the reconstructed and original logMel spectrograms. We transferred our prediction back into an audible waveform by applying a Wavenet vocoder. The vocoder was conditioned on logMel features that harnessed a much larger, pre-existing data corpus to provide the most natural acoustic output. Significance. To the best of our knowledge, this is the first time that high-quality speech has been reconstructed from neural recordings during speech production using deep neural networks.
Interpretation of convolutional neural networks for speech spectrogram regression from intracranial recordings
NeurocomputingM Angrick, C Herff, G Johnson, J Shih, DJ Krusienski, T Schultz
2018-05-19
The direct synthesis of continuously spoken speech from neural activity could provide a fast and natural way of communication for users suffering from speech disorders. Mapping the complex dynamics of neural activity to spectral representations of speech is a demanding task for regression models. Convolutional neural networks have recently shown promise for finding patterns in neural signals and might be a good candidate for this particular regression task. However, the intrinsic agency of the resulting networks is challenging to interpret and thus provides little opportunity to gain insights on neural processes underlying speech. While activation maximization can be used to get a glimpse into what a network has learned for a classification task, it usually does not benefit regression problems.
Here, we show that convolutional neural networks can be used to reconstruct an audible waveform from invasively-measured brain activity. By adapting activation maximization, we present a method that can provide insights from neural networks targeting regression problems. Based on experimental data, we achieve statistically significant correlations between spectrograms of synthesized and original speech. Our interpretation approach shows that trained models reveal that electrodes placed in cortical regions associated with speech production tasks have a large impact on the reconstruction of speech segments.
Biosignal-based Spoken Communication: A Survey
IEEE Trans. Audio, Speech and Language ProcessingT Schultz, M Wand,T Hueber, DJ Krusienski, C Herff, JS Brumberg
Speech is a complex process involving a wide range of biosignals, including but not limited to acoustics. These biosignals-stemming from the articulators, the articulator muscle activities, the neural pathways, and the brain itself-can be used to circumvent limitations of conventional speech processing in particular, and to gain insights into the process of speech production in general. Research on biosignal-based speech processing is a wide and very active field at the intersection of various disciplines, ranging from engineering, computer science, electronics and machine learning to medicine, neuroscience, physiology, and psychology. Consequently, a variety of methods and approaches have been used to investigate the common goal of creating biosignal-based speech processing devices for communication applications in everyday situations and for speech rehabilitation, as well as gaining a deeper understanding of spoken communication. This paper gives an overview of the various modalities, research approaches, and objectives for biosignal-based spoken communication.
Optimization of Checkerboard Spatial Frequencies for Steady-State Visual Evoked Potential Brain-Computer Interfaces
IEEE Trans Neural Syst Rehabil Eng.NR Waytowich, Y Yamani, DJ Krusienski
Steady-state visual evoked potentials (SSVEPs) are oscillations of the electroencephalogram (EEG) which are mainly observed over the occipital area that exhibit a frequency corresponding to a repetitively flashing visual stimulus. SSVEPs have proven to be very consistent and reliable signals for rapid EEG-based brain-computer interface (BCI) control. There is conflicting evidence regarding whether solid or checkerboard-patterned flashing stimuli produce superior BCI performance. Furthermore, the spatial frequency of checkerboard stimuli can be varied for optimal performance. The present study performs an empirical evaluation of performance for a 4-class SSVEP-based BCI when the spatial frequency of the individual checkerboard stimuli is varied over a continuum ranging from a solid background to single-pixel checkerboard patterns. The results indicate that a spatial frequency of 2.4 cycles per degree can maximize the information transfer rate with a reduction in subjective visual irritation compared to lower spatial frequencies. This important finding on stimulus design can lead to improved performance and usability of SSVEP-based BCIs.
Spatio-Temporal Progression of Cortical Activity Related to Continuous Overt and Covert Speech Production in a Reading Task
PLOS ONEJS Brumberg, DJ Krusienski, S Chakrabarti, A Gunduz, P Brunner, AL Ritaccio, G Schalk
2016-11-22
How the human brain plans, executes, and monitors continuous and fluent speech has remained largely elusive. For example, previous research has defined the cortical locations most important for different aspects of speech function, but has not yet yielded a definition of the temporal progression of involvement of those locations as speech progresses either overtly or covertly. In this paper, we uncovered the spatio-temporal evolution of neuronal population-level activity related to continuous overt speech, and identified those locations that shared activity characteristics across overt and covert speech. Specifically, we asked subjects to repeat continuous sentences aloud or silently while we recorded electrical signals directly from the surface of the brain (electrocorticography (ECoG)). We then determined the relationship between cortical activity and speech output across different areas of cortex and at sub-second timescales. The results highlight a spatio-temporal progression of cortical involvement in the continuous speech process that initiates utterances in frontal-motor areas and ends with the monitoring of auditory feedback in superior temporal gyrus. Direct comparison of cortical activity related to overt versus covert conditions revealed a common network of brain regions involved in speech that may implement orthographic and phonological processing. Our results provide one of the first characterizations of the spatiotemporal electrophysiological representations of the continuous speech process, and also highlight the common neural substrate of overt and covert speech. These results thereby contribute to a refined understanding of speech functions in the human brain