Spotlight
Areas of Expertise (7)
AI and Machine Learning
Applied Machine Learning (e.g. Medical Computer Vision)
Language-guided Scene Understanding
Artificial Intelligence
Deep Learning
Medical Computer Vision
AI
Media
Publications:
Documents:
Photos:
Audio/Podcasts:
Links (2)
Biography
Christopher Kanan is a tenured associate professor of computer science. His main research focus is deep learning, with an emphasis on lifelong (continual) machine learning, bias-robust artificial intelligence, medical computer vision, and language-guided scene understanding. He has worked on online continual learning, visual question answering, computational pathology, self-supervised learning, semantic segmentation, object recognition, object detection, active vision, object tracking, and more. Beyond machine learning, he has a background in eye tracking, primate vision, and theoretical neuroscience.
He is on the Scientific Advisory Board (SAB) of Paige.AI, Inc. whose goal is to revolutionize pathology and oncology by creating clinical-grade AI systems to help pathologists and to predict treatment relevant computational biomarkers.
Prof. Kanan was previously affiliated with NASA Jet Propulsion Laboratory (JPL).
Education (3)
University of California, San Diego: PhD, Computer Science 2013
University of Southern California: MS, Computer Science 2006
Oklahoma State University: BS, Philosophy and Computer Science 2004
Selected Media Appearances (4)
Can we teach AI to learn like humans?
Academic Minute WAMC radio
2025-02-19
On University of Rochester Week: Human intelligence and artificial intelligence learn differently, but can that change? Chris Kanan, associate professor of computer science at the Hajim School of Engineering and Computer Science, looks at the possibilities. Christopher Kanan’s main research focus is deep learning, with an emphasis on lifelong (continual) machine learning, bias-robust artificial intelligence, medical computer vision, and language-guided scene understanding. Can we teach AI to learn like humans? Artificial intelligence algorithms don’t learn like people. Instead of continuously updating their knowledge base with new information over time as humans do, algorithms learn only during their training phase. After that, their knowledge remains frozen; they perform the task they were trained for without being able to keep learning as they do it. Learning new information often requires trained the system again from scratch; otherwise, systems can suffer from catastrophic forgetting, where they incorporate new knowledge at the cost of forgetting nearly everything it’s already learned. This situation arises because of the way that today’s most powerful AI algorithms, called neural networks, learn new things. To help counter this, I have helped establish a new field of AI research known as continual learning. The goal is to keep AI learning new things from continuous streams of data, and to do so without forgetting everything that came before. This is a fundamental thing that we need to solve to make artificial general intelligence one day.
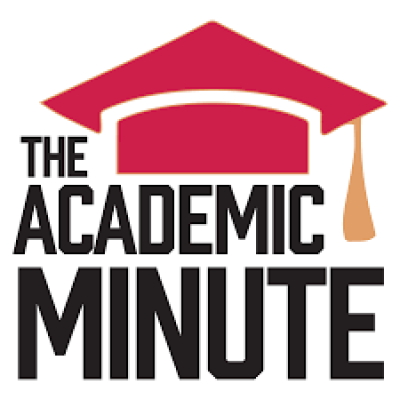
Leveraging AI in the workplace and education brings both pros and cons
Rochester Business Journal print
2024-05-01
“It doesn’t know what’s false, what’s right, it just knows given what you’ve asked it to do or what you’ve written, what’s the next word it should say,” said Chris Kanan, associate professor of computer science at the University of Rochester’s Center for Visual Science, Brain & Cognitive Sciences.
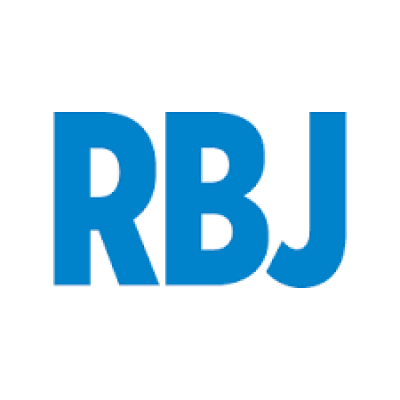
AI's Rising Comes with No Easy Answers
Rochester Beacon online
2023-05-23
The May 23 event featured Pencheng Shi, associate dean at RIT’s Golisano College of Computing and Information Sciences; Chris Kanan, associate professor of computer science at the University of Rochester; and Tim Madigan, professor and chair of philosophy at St. John Fisher University. “(AI) has been everywhere over the past few years (but) has been less obvious. Unlocking your phone with your face, that’s AI,” Kanan said.
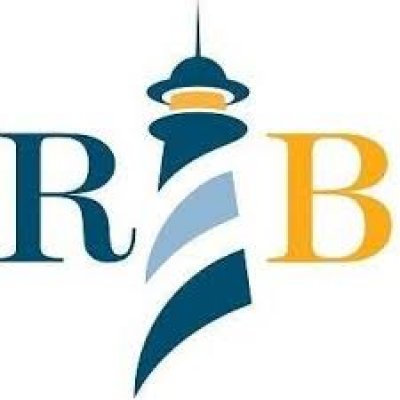
The Computer Scientist Challenging AI to Learn Better
Quanta Magazine online
2022-08-02
Christopher Kanan is building algorithms that can continuously learn over time — the way we do.
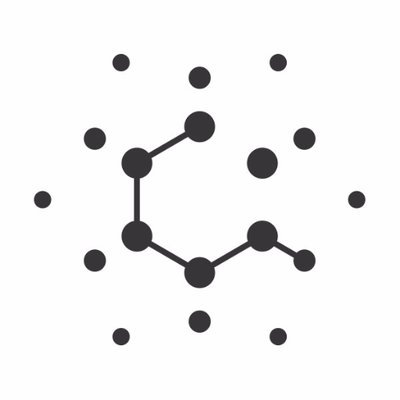
Patents (5)
Systems and methods to process electronic images to adjust attributes of the electronic images
11,455,753
2022-09-27
Systems and methods to process electronic images to adjust attributes of the electronic images.
Systems and methods to process electronic images to provide image-based cell group targeting
11,436,727
2022-09-06
Systems and methods to process electronic images to provide image-based cell group targeting.
Systems and methods to process electronic images to provide localized semantic analysis of whole slide images
11,430,117
2022-08-30
Systems and methods to process electronic images to provide localized semantic analysis of whole slide images.
Systems and methods to process electronic images to provide localized semantic analysis of whole slide images
11,430,116
2022-08-30
Systems and methods to process electronic images to provide localized semantic analysis of whole slide images.
Systems and methods for processing electronic images for computational detection methods
11,423,547
2022-08-23
Systems and methods for processing electronic images for computational detection methods.
Selected Articles (5)
Clinical Validation of Artificial Intelligence Augmented Pathology Diagnosis Demonstrates Significant Gains in Diagnostic Accuracy in Prostate Cancer Detection.
Archives of Pathology & Laboratory MedicineChristopher Kanan (and 19 others)
2022-12-20
Prostate cancer diagnosis rests on accurate assessment of tissue by a pathologist. The application of artificial intelligence (AI) to digitized whole slide images (WSIs) can aid pathologists in cancer diagnosis, but robust, diverse evidence in a simulated clinical setting is lacking.
Replay in Deep Learning: Current Approaches and Missing Biological Elements
Neural ComputationChristopher Kanan, Tyler L. Hayes, Giri P. Krishnan, Maxim Bazhenov, Hava T. Siegelmann, and Terrence Sejnowski
2021-05-28
Replay is the reactivation of one or more neural patterns, which are similar to the activation patterns experienced during past waking experiences. Replay was first observed in biological neural networks during sleep, and it is now thought to play a critical role in memory formation, retrieval, and consolidation. Replay-like mechanisms have been incorporated into deep artificial neural networks that learn over time to avoid catastrophic forgetting of previous knowledge. Replay algorithms have been successfully used in a wide range of deep learning methods within supervised, unsupervised, and reinforcement learning paradigms. In this paper, we provide the first comprehensive comparison between replay in the mammalian brain and replay in artificial neural networks. We identify multiple aspects of biological replay that are missing in deep learning systems and hypothesize how they could be utilized to improve artificial neural networks.
Detecting Spurious Correlations with Sanity Tests for Artificial Intelligence Guided Radiology Systems
Frontiers in Digital HealthChristopher Kanan, Usman Mahmood, Robik Shrestha, David D. B. Bates, Lorenzo Mannelli, Giuseppe Corrias, and Yusuf Emre Erdi
2021-08-03
Artificial intelligence (AI) has been successful at solving numerous problems in machine perception. In radiology, AI systems are rapidly evolving and show progress in guiding treatment decisions, diagnosing, localizing disease on medical images, and improving radiologists' efficiency. A critical component to deploying AI in radiology is to gain confidence in a developed system's efficacy and safety. The current gold standard approach is to conduct an analytical validation of performance on a generalization dataset from one or more institutions, followed by a clinical validation study of the system's efficacy during deployment. Clinical validation studies are time-consuming, and best practices dictate limited re-use of analytical validation data, so it is ideal to know ahead of time if a system is likely to fail analytical or clinical validation. In this paper, we describe a series of sanity tests to identify when a system performs well on development data for the wrong reasons. We illustrate the sanity tests' value by designing a deep learning system to classify pancreatic cancer seen in computed tomography scans.
Quality control of radiomic features using 3D printed CT phantoms
Journal of Medical ImagingChristopher Kanan, Usman Mahmood, Aditya Apte, David D. B. Bates, Giuseppe Corrias, Lorenzo Mannelli, Jung Hun Oh, Yusuf Emre Erdi, John Nguyen, Joseph O'Deasy, and Amita Shukla-Dave
2021-06-29
The lack of standardization in quantitative radiomic measures of tumors seen on computed tomography (CT) scans is generally recognized as an unresolved issue. To develop reliable clinical applications, radiomics must be robust across different CT scan modes, protocols, software, and systems. We demonstrate how custom-designed phantoms, imprinted with human-derived patterns, can provide a straightforward approach to validating longitudinally stable radiomic signature values in a clinical setting.
Independent real-world application of a clinical-grade automated prostate cancer detection system
Journal of PathologyChristopher Kanan (and 20 others)
2021-04-27
Artificial intelligence (AI)-based systems applied to histopathology whole-slide images have the potential to improve patient care through mitigation of challenges posed by diagnostic variability, histopathology caseload, and shortage of pathologists. We sought to define the performance of an AI-based automated prostate cancer detection system, Paige Prostate, when applied to independent real-world data.
Social