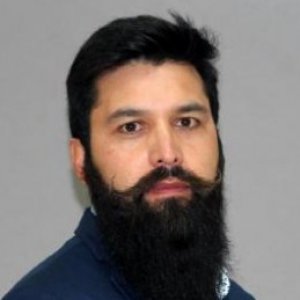
Ernesto Guerra Vallejos, Ph.D.
Adjunct Assistant Professor Milwaukee School of Engineering
- Milwaukee WI
Ernesto Guerra Vallejois is an economist, data analytics director, research scientist, statistician, and professor.
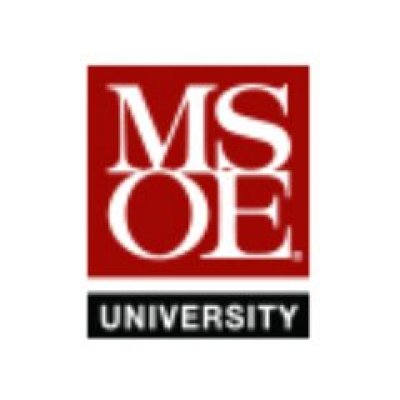
Milwaukee School of Engineering
View more experts managed by Milwaukee School of Engineering
Education, Licensure and Certification
Ph.D.
Agricultural & Resource Economics
University of California, Berkeley
2018
M.S.
Agricultural & Resource Economics
University of California, Berkeley
2013
M.S.
Environmental & Natural Resource Economics
Universidad de Concepción
2012
B.Econ.
Economics
Universidad de Concepción
2012
Areas of Expertise
Accomplishments
Scientific Productivity Award, Economics Department, Universidad Católica de la Santísima Concepción
2021, 2022
Summer School Grant,“Thematic Semester on Commodity Derivatives Markets”
2015
World Bank Conference Grant,“Causes and Types of Price Volatility”
2014
Fulbright Program Fellowship
2012 - 2016
Affiliations
- Agricultural & Applied Economics Association (AAEA) : Member
- Journal of Business & Economic Statistics : Reviewer
- Food Policy : Reviewer
- new Initiative for Computational Economics (nICE) : Speaker
Social
Selected Publications
Endogenous thresholds in energy prices: Modeling and empirical estimation☆
Energy Economics2023
Spot prices or front-month futures prices for energy commodities can exhibit occasional sharp peaks and drops (even to negative values), accompanied by large backwardations or contangos and unusually low or high levels of working stocks. We present a model of storage arbitrage that rationalizes such episodes, relevant for markets for energy commodities such as petroleum, natural gas, or storable electricity, where free disposal is not available.
Solving dynamic stochastic models with multiple occasionally binding constraints☆
Economic Modelling2021
Non-negativity and capacity constraints on accumulation, and price floors, have particular relevance for water, oil, gas, electricity, and other energy commodities. Such constraints are empirically relevant; even if historical records do not include periods where the capacity constraint is binding or where marginal value is zero, a positive probability that such events might occur affects rational accumulation and consumption decisions.
Putting the Empirical Commodity Storage Model Back on Track: Crucial Implications of a “Negligible” Trend
American Journal of Agricultural Economics2020
The dynamics of consumption and stocks are crucial for analysis of commodity prices and policies. But empirical application of the standard storage model has been derailed by failure to replicate high real price autocorrelation. Our proposed storage model is the first empirical model to recognize the full implications of nonstationarity for price behavior and speculative arbitrage with an occasionally binding non-negativity constraint, a challenge shared by DSGE models in macroeconomics and growth.
Empirical commodity storage model: the challenge of matching data and theory
European Review of Agricultural Economics2014
The ability of the standard commodity storage model to replicate annual price serial correlation is a controversial issue. Calendar year averages of prices induce spurious smoothing of price spikes, a fact that has been surprisingly overlooked in several empirical estimations of the annual commodity storage model for agricultural commodities. We present the application of a maximum likelihood estimator of the storage model for maize prices, correcting for the spurious smoothing. We find, for this data set, serious differences in magnitudes of interest.
Data-Driven Condition Monitoring of Mining Mobile Machinery in Non-Stationary Operations Using Wireless Accelerometer Sensor Modules.
IEEE Access2013
This paper presents the development of an easy-to-deploy and smart monitoring IoT system that utilizes vibration measurement devices to assess real-time condition of bulldozers, power shovels and backhoes, in non-stationary operations in the mining industry. According to operating experience data and the type of mining machine, total loss failure rates per machine fleet can reach up to 30%. Vibration analysis techniques are commonly used for condition monitoring and early detection of unforeseen failures to generate predictive maintenance plans for heavy machinery.