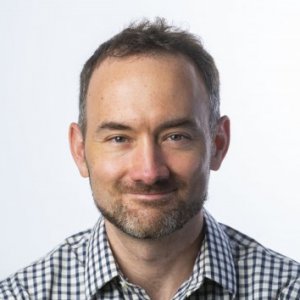
Graham Neubig
Associate Professor Carnegie Mellon University
- Pittsburgh PA
Graham Neubig's research is concerned with language and its role in human communication.
Biography
Areas of Expertise
Media Appearances
AI isn't ready to do your job
Business Insider online
2025-04-22
AI Agents aren't ready to do your job. Researchers at CMU staffed a fake company with AI agents, and the results were disastrous. "While agents may be used to accelerate some portion of the tasks that human workers are doing, they are likely not a replacement for all tasks at the moment," said Graham Neubig (School of Computer Science).
Where DeepL Beats ChatGPT in Machine Translation with Graham Neubig
Slator online
2023-07-14
In this week’s SlatorPod, we are joined by Graham Neubig, Associate Professor of Computer Science at Carnegie Mellon University, to discuss his research on multilingual natural language processing (NLP) and machine translation (MT).
Angry Bing chatbot just mimicking humans, say experts
ARY News online
2023-02-18
“I think this is basically mimicking conversations that it’s seen online,” said Graham Neubig, an associate professor at Carnegie Mellon University’s language technologies institute.
The Latest in Translation Devices
The New York Times online
2019-11-07
These devices “bring us a bit closer to being able to travel to places in the world where people speak different languages and communicate smoothly with those who are living there,” said Graham Neubig, an assistant professor at the Language Technologies Institute of Carnegie Mellon University and an expert in machine learning and natural language processing.
Social
Industry Expertise
Education
Kyoto University
Ph.D.
Informatics
2012
Kyoto University
M.S.
Informatics
2010
University of Illinois Urbana-Champaign
B.S.
Computer Science
2005
Articles
Divergences between Language Models and Human Brains
Advances in Neural Information Processing Systems2024
Do machines and humans process language in similar ways? Recent research has hinted at the affirmative, showing that human neural activity can be effectively predicted using the internal representations of language models (LMs). Although such results are thought to reflect shared computational principles between LMs and human brains, there are also clear differences in how LMs and humans represent and use language. In this work, we systematically explore the divergences between human and machine language processing by examining the differences between LM representations and human brain responses to language as measured by Magnetoencephalography (MEG) across two datasets in which subjects read and listened to narrative stories. Using an LLM-based data-driven approach, we identify two domains that LMs do not capture well: social/emotional intelligence and physical commonsense.
Do llms exhibit human-like response biases? a case study in survey design
Transactions of the Association for Computational Linguistics2024
One widely cited barrier to the adoption of LLMs as proxies for humans in subjective tasks is their sensitivity to prompt wording—but interestingly, humans also display sensitivities to instruction changes in the form of response biases. We investigate the extent to which LLMs reflect human response biases, if at all. We look to survey design, where human response biases caused by changes in the wordings of “prompts” have been extensively explored in social psychology literature. Drawing from these works, we design a dataset and framework to evaluate whether LLMs exhibit human-like response biases in survey questionnaires. Our comprehensive evaluation of nine models shows that popular open and commercial LLMs generally fail to reflect human-like behavior, particularly in models that have undergone RLHF.
DIRE and its data: Neural decompiled variable renamings with respect to software class
ACM Transactions on Software Engineering and Methodology2023
The decompiler is one of the most common tools for examining executable binaries without the corresponding source code. It transforms binaries into high-level code, reversing the compilation process. Unfortunately, decompiler output is far from readable because the decompilation process is often incomplete. State-of-the-art techniques use machine learning to predict missing information like variable names. While these approaches are often able to suggest good variable names in context, no existing work examines how the selection of training data influences these machine learning models. We investigate how data provenance and the quality of training data affect performance, and how well, if at all, trained models generalize across software domains. We focus on the variable renaming problem using one such machine learning model, DIRE.
AmericasNLI: Machine translation and natural language inference systems for Indigenous languages of the Americas
Frontiers in Artificial Intelligence2022
Little attention has been paid to the development of human language technology for truly low-resource languages—i.e., languages with limited amounts of digitally available text data, such as Indigenous languages. However, it has been shown that pretrained multilingual models are able to perform crosslingual transfer in a zero-shot setting even for low-resource languages which are unseen during pretraining. Yet, prior work evaluating performance on unseen languages has largely been limited to shallow token-level tasks. It remains unclear if zero-shot learning of deeper semantic tasks is possible for unseen languages. To explore this question, we present AmericasNLI, a natural language inference dataset covering 10 Indigenous languages of the Americas.
Can we automate scientific reviewing?
Journal of Artificial Intelligence Research2022
The rapid development of science and technology has been accompanied by an exponential growth in peer-reviewed scientific publications. At the same time, the review of each paper is a laborious process that must be carried out by subject matter experts. Thus, providing high-quality reviews of this growing number of papers is a significant challenge. In this work, we ask the question “can we automate scientific reviewing?”, discussing the possibility of using natural language processing (NLP) models to generate peer reviews for scientific papers. Because it is non-trivial to define what a “good” review is in the first place, we first discuss possible evaluation metrics that could be used to judge success in this task.