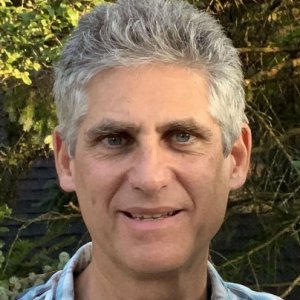
Michael Tarr
Professor and Department Head Carnegie Mellon University
- Pittsburgh PA
Michael Tarr is an expert in visual perception and how the brain transforms 2D images into high-level percepts.
Biography
Areas of Expertise
Media Appearances
CMU startup Neon honored by World Economic Forum
The Business Journals online
2015-08-05
Neon was co-founded by Michael Tarr, head of the Psychology Department in CMU's Dietrich College of Humanities and Social Science, and Sophie Lebrecht, who received her postdoctoral training at CMU.
Social
Industry Expertise
Accomplishments
Fellow, American Association for the Advancement of Science (AAAS
2017
Education
Massachusetts Institute of Technology
Ph.D.
Brain and Cognitive Sciences
Cornell University
B.S.
Psychology
Links
Articles
Low-level tuning biases in higher visual cortex reflect the semantic informativeness of visual features
Journal of Vision2023
Representations of visual and semantic information can overlap in human visual cortex, with the same neural populations exhibiting sensitivity to low-level features (orientation, spatial frequency, retinotopic position) and high-level semantic categories (faces, scenes). It has been hypothesized that this relationship between low-level visual and high-level category neural selectivity reflects natural scene statistics, such that neurons in a given category-selective region are tuned for low-level features or spatial positions that are diagnostic of the region's preferred category. To address the generality of this “natural scene statistics” hypothesis, as well as how well it can account for responses to complex naturalistic images across visual cortex, we performed two complementary analyses.
A texture statistics encoding model reveals hierarchical feature selectivity across human visual cortex
Perception2023
Midlevel features, such as contour and texture, provide a computational link between low- and high-level visual representations. Although the nature of midlevel representations in the brain is not fully understood, past work has suggested a texture statistics model, called the P–S model , is a candidate for predicting neural responses in areas V1–V4 as well as human behavioral data. However, it is not currently known how well this model accounts for the responses of higher visual cortex to natural scene images. To examine this, we constructed single-voxel encoding models based on P–S statistics and fit the models to fMRI data from human subjects (both sexes) from the Natural Scenes Dataset.
Selectivity for food in human ventral visual cortex
Communications Biology2023
Visual cortex contains regions of selectivity for domains of ecological importance. Food is an evolutionarily critical category whose visual heterogeneity may make the identification of selectivity more challenging. We investigate neural responsiveness to food using natural images combined with large-scale human fMRI. Leveraging the improved sensitivity of modern designs and statistical analyses, we identify two food-selective regions in the ventral visual cortex. Our results are robust across 8 subjects from the Natural Scenes Dataset (NSD), multiple independent image sets and multiple analysis methods. We then test our findings of food selectivity in an fMRI “localizer” using grayscale food images.
Brain Dissection: fMRI-trained Networks Reveal Spatial Selectivity in the Processing of Natural Images
bioRxiv2023
The alignment between deep neural network (DNN) features and cortical responses currently provides the most accurate quantitative explanation for higher visual areas. At the same time, these model features have been critiqued as uninterpretable explanations, trading one black box (the human brain) for another (a neural network). In this paper, we train networks to directly predict, from scratch, brain responses to images from a large-scale dataset of natural scenes. We then employ "network dissection" (Bau et al., 2017), a method used for enhancing neural network interpretability by identifying and localizing the most significant features in images for individual units of a trained network, and which has been used to study category selectivity in the human brain (Khosla & Wehbe, 2022). We adapt this approach to create a hypothesis-neutral model that is then used to explore the tuning properties of specific visual regions beyond category selectivity, which we call "brain dissection".
Early experience with low-pass filtered images facilitates visual category learning in a neural network model
PLoS ONE2023
Humans are born with very low contrast sensitivity, meaning that inputs to the infant visual system are both blurry and low contrast. Is this solely a byproduct of maturational processes or is there a functional advantage for beginning life with poor visual acuity? We addressed the impact of poor vision during early learning by exploring whether reduced visual acuity facilitated the acquisition of basic-level categories in a convolutional neural network model (CNN), as well as whether any such benefit transferred to subordinate-level category learning. Using the ecoset dataset to simulate basic-level category learning, we manipulated model training curricula along three dimensions: presence of blurred inputs early in training, rate of blur reduction over time, and grayscale versus color inputs. First, a training regime where blur was initially high and was gradually reduced over time—as in human development—improved basic-level categorization performance in a CNN relative to a regime in which non-blurred inputs were used throughout training.