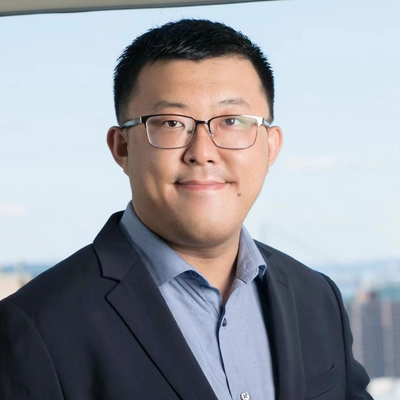
Mingzhang Yin
Assistant Professor University of Florida
- Gainesville FL
Mingzhang Yin studies probabilistic machine learning, Bayesian methods and causal inference.
Biography
Areas of Expertise
Media Appearances
Building New Tools at the Intersection of Statistical Machine Learning and Causal Inference
Columbia University Data Science Institute online
2022-04-14
Data Science Institute (DSI) and Irving Institute for Cancer Dynamics postdoctoral research scientist Mingzhang Yin focuses on problems related to machine learning, Bayesian statistics, and causal inference. And as a future professor, he excels at clearly describing such complex subject matter.
Social
Articles
Probabilistic Machine Learning: New Frontiers for Modeling Consumers and their Choices
International Journal of Research in MarketingDew, et al.
2024-11-11
Making sense of massive, individual-level data is challenging: marketing researchers and analysts need flexible models that can accommodate rich patterns of heterogeneity and dynamics, work with and link diverse data types, and scale to modern data sizes. Practitioners also need tools that can quantify uncertainty in models and predictions of consumer behavior to inform optimal decision-making.
Optimization-based Causal Estimation from Heterogeneous Environments
Journal of Machine Learning ResearchYin, et al.
2024-04-01
This paper presents a new optimization approach to causal estimation. Given data that contains covariates and an outcome, which covariates are causes of the outcome, and what is the strength of the causality? In classical machine learning (ML), the goal of optimization is to maximize predictive accuracy. However, some covariates might exhibit a non-causal association with the outcome. Such spurious associations provide predictive power for classical ML, but they prevent us from causally interpreting the result.
Conformal Sensitivity Analysis for Individual Treatment Effects
Journal of the American Statistical AssociationYin, et al.
2021-12-12
Estimating an individual treatment effect (ITE) is essential to personalized decision making. However, existing methods for estimating the ITE often rely on unconfoundedness, an assumption that is fundamentally untestable with observed data. To assess the robustness of individual-level causal conclusion with unconfoundedness, this article proposes a method for sensitivity analysis of the ITE, a way to estimate a range of the ITE under unobserved confounding.