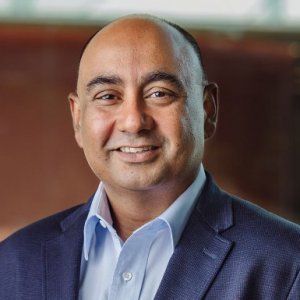
Param Singh
Professor of Business Technologies and Marketing Carnegie Mellon University
- Pittsburgh PA
Param Singh's research focuses on the intersection of economics, machine learning, and AI.
Biography
Areas of Expertise
Media Appearances
Study unveils the career impact of attractiveness: Higher salaries and prestigious roles over time
Phys Org online
2025-01-27
"This research underscores how biases tied to physical appearance persist in shaping career outcomes, even for highly educated professionals," says Param Vir Singh, co-author and professor at Carnegie Mellon University (CMU).
Move over Bitcoin. There's a new cryptocurrency in town
Pittsburgh Post-Gazette online
2018-05-18
Experts at CMU in economics, finance, accounting, consumer behavior and privacy are working to improve blockchain technology and policy surrounding crypto, said Param Vir Singh, an associate professor of business technologies at CMU’s Tepper School of Business.
Social
Industry Expertise
Accomplishments
Finalist
2023 Don Morrison Long Term Impact Award in Marketing
Distinguished Fellow
2019 INFORMS Information Systems Society
Service Award
2017 Information Systems Research
Education
University of Washington
Ph.D.
Information Systems
2008
Panjab University
B.S.
Chemical Engineering
2000
Articles
Personalization, Consumer Search and Algorithmic Pricing
Marketing Science2025
Our study investigates the impact of product ranking systems on artificial intelligence (AI) powered pricing algorithms. Specifically, we examine the effects of "personalized" and "unpersonalized" ranking systems on algorithmic pricing outcomes and consumer welfare. Our analysis reveals that personalized ranking systems, which rank products in decreasing order of consumer's utilities, may encourage higher prices charged by pricing algorithms, especially when consumers search for products sequentially on a third-party platform. This is because personalized ranking significantly reduces the ranking-mediated price elasticity of demand and thus incentives to lower prices. Conversely, unpersonalized ranking systems lead to significantly lower prices and greater consumer welfare. These findings suggest that even in the absence of price discrimination, personalization may not necessarily benefit consumers since pricing algorithms can undermine consumer welfare through higher prices. Thus, our study highlights the crucial role of ranking systems in shaping algorithmic pricing behaviors and consumer welfare.
Unequal Impact of Zestimate on the Housing Market
Marketing Science2025
We study the impact of Zillow’s Zestimate on housing market outcomes and how the impact differs across socio-economic segments. Zestimate is produced by a Machine Learning algorithm using large amounts of data and aims to predict a home’s market value at any time. Zestimate can potentially help market participants in the housing market as identifying the value of a home is a non-trivial task. However, inaccurate Zestimate could also lead to incorrect beliefs about property values and therefore suboptimal decisions, which would hinder the selling process. Meanwhile, Zestimate tends to be systematically more accurate for rich neighborhoods than poor neighborhoods, raising concerns that the benefits of Zestimate may accrue largely to the rich, which could widen socio-economic inequality. Using data on Zestimate and housing sales in the United States, we show that Zestimate overall benefits the housing market, as on average it increases both buyer surplus and seller profit. This is primarily because its uncertainty reduction effect allows sellers to be more patient and set higher reservation prices to wait for buyers who truly value the properties, which improves seller-buyer match quality. Moreover, Zestimate actually reduces socio-economic inequality, as our results reveal that both rich and poor neighborhoods benefit from Zestimate but the poor neighborhoods benefit more. This is because poor neighborhoods face greater prior uncertainty and therefore would benefit more from new signals.
Do Lower-Quality Images Lead to Higher Demand on Airbnb?
SSRN Electronic Journal2023
Prior research has shown that high-quality images increase the current demand for Airbnb properties. However, many properties do not adopt high-quality images even when offered for free by Airbnb. Our study provides an answer to this puzzling observation. We develop a structural model of demand and supply of Airbnb properties, where hosts strategically choose image quality for their properties. Using a one-year panel data from 958 properties in Manhattan, we find evidence that a host’s decision to use high-quality images entails a trade-off: high-quality images may attract more guests in the current period, but if the property does not live up to the expectations created by the image quality, then they risk disappointing guests. The guests would then leave bad no reviews at all, which would adversely affect future demand. Counterfactual policy simulations show that Airbnb could significantly increase its profits (up to 18.9%) by offering medium-quality images for free to hosts or providing free access to a choice between high-quality and medium-quality images. These policies help improve Airbnb's profits since they enable the hosts to upgrade their image quality to an extent that aligns with their property quality.
Consumer Risk Preferences Elicitation From Large Language Models
SSRN Electronic Journal2023
Our study evaluates Large Language Models (LLMs), like GPT-4, for their ability to mimic consumer-revealed preferences in risk-influenced decision-making. We compared LLMs' predictions to actual consumer choices when selecting home insurance plans. Findings reveal that LLMs closely match consumer decisions on an aggregate but not individual level. We propose a "Theory-based Chain-of-Verification" approach to examine if LLMs outcomes align with consumer risk preferences. We find that LLMs display a tendency towards "extremeness aversion" in multi-option scenarios, reflecting a rudimentary decision-making approach rather than a nuanced understanding of consumer risk preferences. Further investigation using binary choice scenarios—to mitigate the extremeness aversion effect—suggests that LLMs are capable of learning risk preferences, albeit with significant limitations. Specifically, LLMs underestimate consumer risk aversion and diverge from actual consumer behaviors in risk preferences. They show diminished sensitivity to losses than gains, with a loss aversion coefficient of 1.09, contrasting sharply with the consumer-derived 2.56. Additionally, LLMs overweigh low-probability risks, evaluating a 4% risk at 15.4%, significantly deviating from the literature-supported 9.7%. Despite these discrepancies, LLMs can offer relatively accurate predictions of expected profit when creating insurance menus. Nonetheless, they exhibit considerable limitations in evaluating the profitability of insurance options designed by humans.
Algorithmic Transparency with Strategic Users
Management Science2023
Should firms that apply machine learning algorithms in their decision making make their algorithms transparent to the users they affect? Despite the growing calls for algorithmic transparency, most firms keep their algorithms opaque, citing potential gaming by users that may negatively affect the algorithm's predictive power. In this paper, we develop an analytical model to compare firm and user surplus with and without algorithmic transparency in the presence of strategic users and present novel insights. We identify a broad set of conditions under which making the algorithm transparent actually benefits the firm. We show that, in some cases, even the predictive power of the algorithmcan increase if the firm makes the algorithm transparent. By contrast, users may not always be better off under algorithmic transparency. These results hold evenwhen the predictive power of the opaque algorithmcomes largely from correlational features and the cost for users to improve them is minimal. We show that these insights are robust under several extensions of the main model. Overall, our results show that firms should not always view manipulation by users as bad. Rather, they should use algorithmic transparency as a lever tomotivate users to invest inmore desirable features.