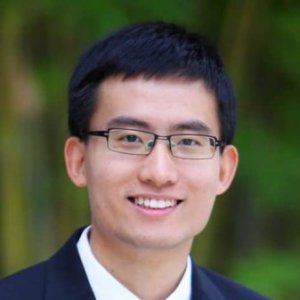
Liangfei Qiu
Professor University of Florida
- Gainesville FL
Liangfei Qiu is an expert in social technology, including social media and social networks, as well as artificial intelligence.
Biography
Areas of Expertise
Media Appearances
Cryptocurrency’s surprising transparency advantage
GMU Costello College of Business online
2024-01-02
As perhaps befits a product of the post-2008 economy, the cryptocurrency space has never known normalcy. In a mere 13 years, crypto went from an untried software innovation of mysterious origin to being touted as the future of investing by major movie stars in Super Bowl commercials. Soon thereafter, of course, came the “crypto winter” of 2022, which began well before, but was surely deepened by, the downfall of FTX and disgraced wunderkind Sam Bankman-Fried.
The impact of real-time feedback in employee reviews
EurekAlert! online
2021-05-20
The study, "Are Traditional Performance Reviews Outdated? An Empirical Analysis on Continuous, Real-time Feedback in the Workplace," was conducted by Michael Rivera and Subodha Kumar from Temple University and Liangfei Qiu from the University of Florida. The authors found that the relationship source (peer, subordinate or supervisor) impacts real-time feedback, which tends to be more critical when it comes from supervisors.
Why Negotiators Should Be on Social Networks
INSEAD Knowledge
2019-07-16
Social ties encourage “best behaviour” in negotiations. If there is a high likelihood you will encounter someone another time in the future, you will naturally be less inclined to deceive them. But since no human is perfect, “bad behaviour” may still happen, even if by mistake. Are people more forgiving when the culprit is a network friend?
Social
Articles
How learning effects influence knowledge contribution in online Q&A community? A social cognitive perspective
Decision Support SystemsChencheng Shi, et al.
2021-08-19
Informative contributions are critical for the healthy development of online Q&A communities, which have gained increasing popularity in solving personalized open-ended problems. However, little is known about whether past contribution behaviors and corresponding community feedbacks received affect the characteristics of subsequent contributions. Drawing upon the social cognitive theory, we examine the learning effects on users' knowledge contribution behaviors.
On the Spillover Effects of Online Product Reviews on Purchases: Evidence from Clickstream Data
Information Systems ResearchYoung Kwark, et al.
2021-05-14
We study the spillover effects of the online reviews of other covisited products on the purchases of a focal product using clickstream data from a large retailer. The proposed spillover effects are moderated by (a) whether the related (covisited) products are complementary or substitutive, (b) the choice of media channel (mobile or personal computer (PC)) used, (c) whether the related products are from the same or a different brand, (d) consumer experience, and (e) the variance of the review ratings.
Environmental Factors in Operations Management: The Impact of Air Quality on Product Demand
Production and Operations ManagementYing Ding, et al.
2021-03-26
The operations management literature has recently begun to analyze how novel data sources help practitioners better understand product demand. We extend this stream of research by analyzing how air quality, a prominent environmental factor that has received little attention in prior studies, can impact product demand. Specifically, we examine how air quality affects the demand for different product color options, and find a greater demand for blue-color product option on air-polluted days (vs. clear days).
Multidimensional Observational Learning in Social Networks: Theory and Experimental Evidence
Information Systems ResearchLiangfei Qiu, et al.
2021-03-24
The prevalence of consumers sharing their purchases on social media platforms (e.g., Instagram and Pinterest) and the use of this information by potential future consumers have substantial implications for online retailing. In this study, we examine how product characteristics and the type of information provider jointly impact purchase decisions in a social network setting. We first propose an analytical observational learning framework integrating the impact of product differentiation and social ties.
Informational Efficiency of Cryptocurrency Markets
SSRNLiangfei Qiu, et al.
2021-06-07
We study the price discovery process of cryptocurrencies that trade in unregulated and fragmented markets and have a non-traditional business model. ICOs exhibit significant inefficiencies with VRs (Variance Ratios) less than 0.7 for up to 5-years from the issue date. IEOs with a similar business model as ICOs but underwritten by exchanges are consistently more efficient than ICOs over 200 days, while IPOs are efficient within 30 days of issue.