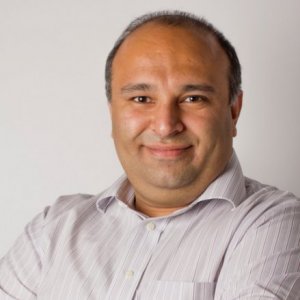
Rayid Ghani
Distinguished Career Professor Carnegie Mellon University
- Pittsburgh PA
Rayid Ghani is a reformed computer scientist who wants to increase the use of large-scale Machine Learning in solving large public policy.
Biography
Rayid is a reformed computer scientist and wanna-be social scientist, but mostly just wants to increase the use of large-scale AI/Machine Learning/Data Science in solving large public policy and social challenges in a fair and equitable manner. Among other areas, Rayid works with governments and non-profits in policy areas such as health, criminal justice, education, public safety, economic development, and urban infrastructure. Rayid is also passionate about teaching practical data science and started the Data Science for Social Good Fellowship that trains computer scientists, statisticians, and social scientists from around the world to work on data science problems with social impact.
Before joining Carnegie Mellon University, Rayid was the Founding Director of the Center for Data Science & Public Policy, Research Associate Professor in Computer Science, and a Senior Fellow at the Harris School of Public Policy at the University of Chicago. Previously, Rayid was the Chief Scientist of the Obama 2012 Election Campaign where he focused on data, analytics, and technology to target and influence voters, donors, and volunteers. In his ample free time, Rayid obsesses over everything related to coffee and works with non-profits to help them with their data, analytics and digital efforts and strategy.
Areas of Expertise
Media Appearances
A Disaster for American Innovation: The Trump administration is jeopardizing the AI boom.
The Atlantic online
2025-04-11
Trump Administration cuts to science puts the U.S. at risk of losing ground as a leader in AI.“I don’t think anybody would seriously claim that these [AI breakthroughs] could have been done if the research universities in the U.S. didn’t exist at the same scale,” said Rayid Ghani (Heinz College).
Artificial Intelligence Makes Energy Demand More Complex — And More Achievable
CMU News online
2025-03-24
The work of individuals like Rayid Ghani, Distinguished Career Professor in the Machine Learning Department and the Heinz College of Information Systems and Public Policy, is one example of how this cross-disciplinary approach can look.
Ghani often looks at the applications of machine learning and artificial intelligence not exclusively in a climate context, but concerning a wide range of social and economic potential. His research primarily focuses on how to use the technology to promote social good in areas such as public health, economic development and urban infrastructure.
The logic behind AI chatbots like ChatGPT is surprisingly basic
Popular Science online
2023-08-22
Systems like ChatGPT can use only what they’ve gleaned from the web. “All it’s doing is taking the internet it has access to and then filling in what would come next,” says Rayid Ghani, a professor in the machine learning department at Carnegie Mellon University.
AI is just another technological step; it won’t exterminate humanity or create a dystopia
The Hill online
2023-07-12
Rayid Ghani co-authored this oped piece on the need to get beyond the hype of AI and examine the facts. "Conflicting narratives describe AI as so powerful it will either extinguish us or revolutionize the quality of life for the whole planet in just the next decade or two. But the awesome power narrative essentially distracts from the real regulatory steps we need to take, today, to rein in the worst actors from benefiting from AI."
How Bias Can Creep into Health Care Algorithms and Data
Discover Magazine online
2022-07-04
Plus, determining the truth of a situation — whether a doctor made a mistake due to poor judgment, racism, or sexism, or whether a doctor just got lucky — isn’t always clear, says Rayid Ghani, a professor in the machine-learning department at Carnegie Mellon University. If a physician runs a test and discovers a patient has diabetes, did the physician do a good job? Yes, they diagnosed the disease. But perhaps they should have tested the patient earlier or treated their rising blood sugar months ago, before the diabetes developed.
Officials seek thousands of poll workers ahead of Election Day, fear shortage due to COVID-19
ABC News online
2020-09-08
Nearly one quarter of counties across eight battleground states are potentially in urgent need of poll workers ahead of the 2020 election, according to a new analysis by Carnegie Mellon University researchers. Rayid Ghani, a professor there, helped create the new tool, which uses historical data to analyze what counties may have the greatest need to help focus recruitment efforts -- but with constantly changing circumstances, it is difficult to predict.
Cities Turn to Software to Predict When Police Will Go Rogue
Bloomberg online
2020-07-15
There are no easy fixes for the Minneapolis Police Department. State lawmakers tried and failed last month to come up with a reform plan after four officers were charged in the death of George Floyd, an unarmed Black man; the city council is proceeding with a proposal to dismantle the department altogether.
The Trump Administration Wants to Regulate Artificial Intelligence
Popular Mechanics online
2020-01-09
Given these are guidelines and not actual policies, the new AI framework is pretty open-ended and non-specific. It's a good start, Rayid Ghani, career professor of machine learning at Carnegie Mellon University's Heinz College, tells Popular Mechanics, but it's imperative that more concrete rules be put into place eventually.
Social
Industry Expertise
Accomplishments
IACP/Laura and John Arnold Foundation Leadership in Law Enforcement Research Award
2018
Milbank Memorial Fund and AcademyHealth State and Local Innovation Prize
2018
American Statistical Association Harry V. Roberts Statistical Advocate of the Year Award
2017
Distinguished Young Alumni Award
2013
Sewanee - University of the South
Education
University of the South
B.S (with Honors)
Computer Science, Mathematics
1999
Carnegie Mellon University
M.S.
Machine Learning
2001
Affiliations
- ChangeLab Solutions : Board of Directors
- The University of the South : Member Board of Regents
- Hispanic Scholarship Fund : Technology Advisor
- AI for Good Foundation : Steering Committee Member
- Data Science for Social Good Foundation : Board Member
- The University of the South : Member Board Of Trustees
Links
Patents
Classification-based redaction in natural language text
US8938386B2
2012-09-12
When redacting natural language text, a classifier is used to provide a sensitive concept model according to features in natural language text and in which the various classes employed are sensitive concepts reflected in the natural language text. Similarly, the classifier is used to provide an utility concepts model based on utility concepts. Based on these models, and for one or more identified sensitive concept and identified utility concept, at least one feature in the natural language text is identified that implicates the at least one identified sensitive topic more than the at least one identified utility concept. At least some of the features thus identified may be perturbed such that the modified natural language text may be provided as at least one redacted document. In this manner, features are perturbed to maximize classification error for sensitive concepts while simultaneously minimizing classification error in the utility concepts.
User modification of generative model for determining topics and sentiments
US9015035B2
2013-01-17
A generative model is used to develop at least one topic model and at least one sentiment model for a body of text. The at least one topic model is displayed such that, in response, a user may provide user input indicating modifications to the at least one topic model. Based on the received user input, the generative model is used to provide at least one updated topic model and at least one updated sentiment model based on the user input. Thereafter, the at least one updated topic model may again be displayed in order to solicit further user input, which further input is then used to once again update the models. The at least one updated topic model and the at least one updated sentiment model may be employed to analyze target text in order to identify topics and associated sentiments therein.
Claims analytics engine
US8762180B2
2014-06-24
Methods and systems for processing claims (e.g., healthcare insurance claims) are described. For example, prior to payment of an unpaid claim, a prediction is made as to whether or not an attribute specified in the claim is correct. Depending on the prediction results, the claim can be flagged for an audit. Feedback from the audit can be used to update the prediction models in order to refine the accuracy of those models.
Articles
Explainable machine learning for public policy: Use cases, gaps, and research directions
Data & Policy2023
Explainability is highly desired in machine learning (ML) systems supporting high-stakes policy decisions in areas such as health, criminal justice, education, and employment. While the field of explainable ML has expanded in recent years, much of this work has not taken real-world needs into account. A majority of proposed methods are designed with generic explainability goals without well-defined use cases or intended end users and evaluated on simplified tasks, benchmark problems/datasets, or with proxy users (e.g., Amazon Mechanical Turk).
Bandit Data-Driven Optimization for Crowdsourcing Food Rescue Platforms
Proceedings of the AAAI Conference on Artificial Intelligence2022
Food waste and insecurity are two societal challenges that coexist in many parts of the world. A prominent force to combat these issues, food rescue platforms match food donations to organizations that serve underprivileged communities, and then rely on external volunteers to transport the food. Previous work has developed machine learning models for food rescue volunteer engagement.
Empirical observation of negligible fairness–accuracy trade-offs in machine learning for public policy
Nature Machine Intelligence2021
The growing use of machine learning in policy and social impact settings has raised concerns over fairness implications, especially for racial minorities. These concerns have generated considerable interest among machine learning and artificial intelligence researchers, who have developed new methods and established theoretical bounds for improving fairness, focusing on the source data, regularization and model training, or post-hoc adjustments to model scores.
An Empirical Comparison of Bias Reduction Methods on Real-World Problems in High-Stakes Policy Settings
ACM SIGKDD Explorations Newsletter2021
Applications of machine learning (ML) to high-stakes policy settings - such as education, criminal justice, healthcare, and social service delivery - have grown rapidly in recent years, sparking important conversations about how to ensure fair outcomes from these systems. The machine learning research community has responded to this challenge with a wide array of proposed fairness-enhancing strategies for ML models, but despite the large number of methods that have been developed, little empirical work exists evaluating these methods in real-world settings.
A recommendation and risk classification system for connecting rough sleepers to essential outreach services
Data & Policy2021
Rough sleeping is a chronic experience faced by some of the most disadvantaged people in modern society. This paper describes work carried out in partnership with Homeless Link (HL), a UK-based charity, in developing a data-driven approach to better connect people sleeping rough on the streets with outreach service providers. HL's platform has grown exponentially in recent years, leading to thousands of alerts per day during extreme weather events; this overwhelms the volunteer-based system they currently rely upon for the processing of alerts.