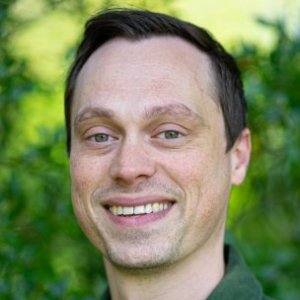
Scott Niekum
Associate Professor, Manning College of Information and Computer Sciences University of Massachusetts Amherst
- Amherst MA
Scott Niekum works to enable personal robots to be deployed in the home and workplace. He is an expert on the social implications of AI.
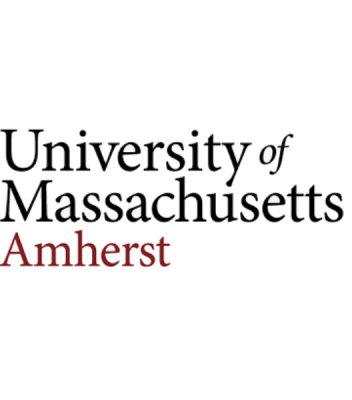
University of Massachusetts Amherst
View more experts managed by University of Massachusetts Amherst
Expertise
Biography
His work draws roughly equally from both machine learning and robotics, including topics such as imitation learning, reinforcement learning, safety, manipulation, and human-robot interaction. Specifically, he is interested in addressing the following questions: How can human demonstrations and interactions be used to bootstrap the learning process? How can robots autonomously improve their understanding of the world through embodied interaction? And how can robots learn from heterogenous, noisy interactions and still provide strong probabilistic guarantees of correctness and safety?
Niekum has also been among the nation's scientists warning about AI and encouraging the imposition of limits.
Social Media
Education
University of Massachusetts Amherst
Ph.D.
Computer Science
University of Massachusetts Amherst
M.S.
Computer Science
Carnegie Mellon University
B.S.
Computer Science
Select Recent Media Coverage
Will artificial intelligence erode our rights?
BBC online
2024-01-26
Scott Niekum discusses the European Union’s AI Act, legislation aiming to maximize the benefits of using AI while protecting our individual rights. "With the emergence of impressive seeming technologies like ChatGPT, I’m really hoping that these tools eventually will blossom into something that can help accelerate scientific discoveries,” he says.
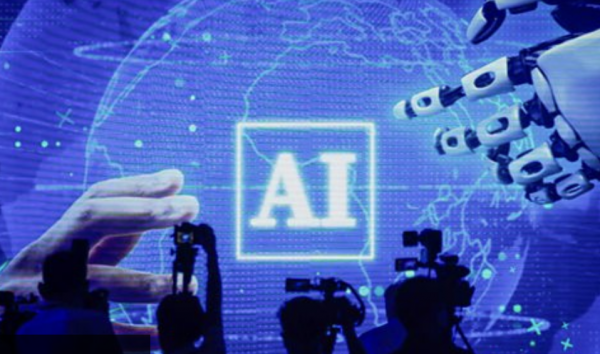
Six Months Ago Elon Musk Called for a Pause on AI. Instead Development Sped Up
Wired online
2023-09-29
Scott Niekum comments in an article on the advancement of AI, despite a letter signed by prominent technology figures to pause advanced AI development. “Many AI skeptics want to hear a concrete doom scenario. To me, the fact that it is difficult to imagine detailed, concrete scenarios is kind of the point—it shows how hard it is for even world-class AI experts to predict the future of AI and how it will impact a complex world. I think that should raise some alarms,” he says.
Sept. 11th: Art and science on the Farms
New England Public Media radio
2023-09-15
Scott Niekum a professor in the UMass Amherst Manning College of Information and Computer Sciences, discusses AI on “The Fabulous 413” radio program and podcast. “A lot about AI right now gives me pause,” Niekum says. “I’m not worried about rogue AIs with crazy, new personalities coming out of nowhere, but what I do worry about is that AI is moving much, much faster than virtually anybody predicted."
Experts issue a dire warning about AI and encourage limits be imposed
NPR online
2023-06-01
Scott Niekum, an associate professor who heads the Safe, Confident, and Aligned Learning + Robotics (SCALAR) lab at the University of Massachusetts Amherst tells NPR's Leila Fadel on Morning Edition that AI has progressed so fast that the threats are still uncalculated, from near-term impacts on minority populations to longer-term catastrophic outcomes. "We really need to be ready to deal with those problems," Niekum said.
Google is poisoning its reputation with AI researchers
The Verge online
2021-04-13
“Not only does it make me deeply question the commitment to ethics and diversity inside the company,” Scott Niekum, an assistant professor at the University of Texas at Austin who works on robotics and machine learning, told The Verge. “But it worries me that they’ve shown a willingness to suppress science that doesn’t align with their business interests.
The Departure of 2 Google AI Researchers Spurs More Fallout
WIRED online
2021-03-16
Another invitee to the event, Scott Niekum, director of a robotics lab at University of Texas at Austin, came to a similar decision. “Google has shown an astounding lack of leadership and commitment to open science, ethics, and diversity in their treatment of the Ethical AI team, specifically Drs. Gebru and Mitchell,” he wrote in his own email to the workshop’s organizers, asking them to pass his decision and comments up to Google’s leadership.
Select Publications
Sope: Spectrum of off-policy estimators
Advances in Neural Information Processing Systems2021
Many sequential decision making problems are high-stakes and require off-policy evaluation (OPE) of a new policy using historical data collected using some other policy. One of the most common OPE techniques that provides unbiased estimates is trajectory based importance sampling (IS). However, due to the high variance of trajectory IS estimates, importance sampling methods based on state-action visitation distributions (SIS) have recently been adopted. Unfortunately, while SIS often provides lower variance estimates for long horizons, estimating the state-action distribution ratios can be challenging and lead to biased estimates.
Adversarial intrinsic motivation for reinforcement learning
Advances in Neural Information Processing Systems2021
Learning with an objective to minimize the mismatch with a reference distribution has been shown to be useful for generative modeling and imitation learning. In this paper, we investigate whether one such objective, the Wasserstein-1 distance between a policy's state visitation distribution and a target distribution, can be utilized effectively for reinforcement learning (RL) tasks. Specifically, this paper focuses on goal-conditioned reinforcement learning where the idealized (unachievable) target distribution has full measure at the goal.
Universal off-policy evaluation
Advances in Neural Information Processing Systems2021
When faced with sequential decision-making problems, it is often useful to be able to predict what would happen if decisions were made using a new policy. Those predictions must often be based on data collected under some previously used decision-making rule. Many previous methods enable such off-policy (or counterfactual) estimation of the expected value of a performance measure called the return. In this paper, we take the first steps towards a'universal off-policy estimator'(UnO)---one that provides off-policy estimates and high-confidence bounds for any parameter of the return distribution.
Understanding the relationship between interactions and outcomes in human-in-the-loop machine learning
International Joint Conference on Artificial Intelligence2021
Human-in-the-loop Machine Learning (HIL-ML) is a widely adopted paradigm for instilling human knowledge in autonomous agents. Many design choices influence the efficiency and effectiveness of such interactive learning processes, particularly the interaction type through which the human teacher may provide feedback. While different interaction types (demonstrations, preferences, etc.) have been proposed and evaluated in the HIL-ML literature, there has been little discussion of how these compare or how they should be selected to best address a particular learning problem.
Importance sampling in reinforcement learning with an estimated behavior policy
Machine Learning2021
In reinforcement learning, importance sampling is a widely used method for evaluating an expectation under the distribution of data of one policy when the data has in fact been generated by a different policy. Importance sampling requires computing the likelihood ratio between the action probabilities of a target policy and those of the data-producing behavior policy. In this article, we study importance sampling where the behavior policy action probabilities are replaced by their maximum likelihood estimate of these probabilities under the observed data. We show this general technique reduces variance due to sampling error in Monte Carlo style estimators.