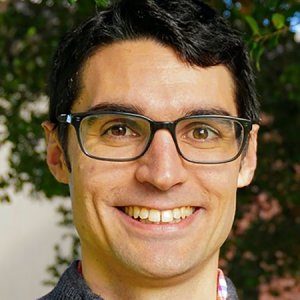
Zachary Manchester
Assistant Professor Carnegie Mellon University
- Pittsburgh PA
Zachary Manchester is a researcher and aerospace engineer with broad interests in dynamics, control, estimation and optimization.
Biography
Areas of Expertise
Media Appearances
This robot dog learned a new trick—balancing like a cat
Popular Science online
2023-04-19
But in robot dogs, their legs aren’t exactly coordinated. If three feet can touch the ground, generally they are fine, but reduce that to one or two robot feet and you’re in trouble. “With current control methods, a quadruped robot’s body and legs are decoupled and don’t speak to one another to coordinate their movements,” Zachary Manchester, an assistant professor in the Robotics Institute and head of the Robotic Exploration Lab, said in a statement. “So how can we improve their balance?”
Quadruped robot uses satellite tools to walk along a balance beam
New Atlas online
2023-04-17
"You basically have a big flywheel with a motor attached," said Manchester. "If you spin the heavy flywheel one way, it makes the satellite spin the other way. Now take that and put it on the body of a quadruped robot."
CMU taught a robot dog to walk a balance beam
TechCrunch online
2023-04-14
“This experiment was huge,” says assistant professor Zachary Manchester. “I don’t think anyone has ever successfully done balance beam walking with a robot before.”
This Massive IKEA-Style Space Station Concept Can Produce Its Own Gravity
Inverse online
2022-03-04
Whether or not this would solve the problems caused by lack of gravity remains to be seen. Still, NASA seems keen on the idea — to the tune of a $600,000 NASA Innovative Advanced Concepts (NIAC) Phase II grant to a team from Carnegie Mellon University (CMU) and the University of Washington (UW) who is looking to develop a structure that can simulate full Earth gravity and be launched in a single rocket.
China ramps up space race with research for giant 1km-long 'gravity' orbiting ship
Daily Express online
2021-11-23
Zachary Manchester, an assistant professor at the robotics institute of Carnegie Mellon University, Pennsylvania, said: "Artificial gravity has been this ‘science fictiony’ holy grail thing for human spaceflight for a century, and the primary way to do it is a large spinning structure."
Social
Industry Expertise
Education
Cornell University
Ph.D.
Aerospace, Aeronautical and Astronautical/Space Engineering
2015
Cornell University
B.S.
Applied Engineering Physics
2009
Affiliations
- American Institute of Aeronautics and Astronautics (AIAA)
Links
Languages
- English
- Spanish
Articles
Propulsion-Free Cross-Track Control of a LEO Small-Satellite Constellation with Differential Drag
2023 62nd IEEE Conference on Decision and Control (CDC)2023
In this work, we achieve propellantless control of both cross-track and along-track separation of a satellite formation by manipulating atmospheric drag. Increasing the differential drag of one satellite with respect to another directly introduces along-track separation, while cross-track separation can be achieved by taking advantage of higher-order terms in the Earth's gravitational field that are functions of altitude. We present an algorithm for solving an n-satellite formation flying problem based on linear programming. We demonstrate this algorithm in a receeding-horizon control scheme in the presence of disturbances and modeling errors in a high-fidelity closed-loop orbital dynamics simulation. Our results show that separation distances of hundreds of kilometers can be achieved by a small-satellite formation in low-Earth orbit over a few months.
Deep Off-Policy Iterative Learning Control
Learning for Dynamics and Control Conference2023
Reinforcement learning has emerged as a powerful paradigm to learn control policies while making few assumptions about the environment. However, this lack of assumptions in popular RL algorithms also leads to sample inefficiency. Furthermore, we often have access to a simulator that can provide approximate gradients for the rewards and dynamics of the environment. Iterative learning control (ILC) approaches have been shown to be very efficient at learning policies by using approximate simulator gradients to speed up optimization. However, they lack the generality of reinforcement learning approaches. In this paper, we take inspiration from ILC and propose an update equation for the value-function gradients (computed using the dynamics Jacobians and reward gradient obtained from an approximate simulator) to speed up value-function and policy optimization.
Practical Critic Gradient based Actor Critic for On-Policy Reinforcement Learning
Learning for Dynamics and Control Conference2023
On-policy reinforcement learning algorithms have been shown to be remarkably efficient at learning policies for continuous control robotics tasks. They are highly parallelizable and hence have benefited tremendously from the recent rise in GPU based parallel simulators. The most widely used on-policy reinforcement learning algorithm is proximal policy optimization (PPO) which was introduced in 2017 and was designed for a somewhat different setting with CPU based serial or less parallelizable simulators. However, suprisingly, it has maintained dominance even on tasks based on the highly parallelizable simulators of today. In this paper, we show that a different class of on-policy algorithms based on estimating the policy gradient using the critic-action gradients are better suited when using highly parallelizable simulators.
Enhanced Balance for Legged Robots Using Reaction Wheels
IEEE International Conference on Robotics and Automation (ICRA)2023
We introduce a reaction wheel system that enhances the balancing capabilities and stability of quadrupedal robots during challenging locomotion tasks. Inspired by both the standard centroidal dynamics model common in legged robotics and models of spacecraft commonly used in the aerospace community, we model the coupled quadruped-reaction-wheel system as a gyrostat, and simplify the dynamics to formulate the problem as a linear discrete-time trajectory optimization problem. Modifications are made to a standard centroidal model-predictive control (MPC) algorithm to solve for both stance foot ground reaction forces and reaction wheel torques simultaneously. The MPC problem is posed as a quadratic program and solved online at 1000 Hz.
Cerberus: Low-Drift Visual-Inertial-Leg Odometry For Agile Locomotion
2023 IEEE International Conference on Robotics and Automation (ICRA)2023
We present an open-source Visual-Inertial-Leg Odometry (VILO) state estimation solution for legged robots, called Cerberus, which precisely estimates position on various terrains in real-time using a set of standard sensors, including stereo cameras, IMU, joint encoders, and contact sensors. In addition to estimating robot states, we perform online kinematic parameter calibration and outlier rejection to substantially reduce position drift. Hardware experiments in various indoor and outdoor environments validate that online calibration of kinematic parameters can reduce estimation drift to less than 1% during long-distance, high-speed locomotion. Our drift results are better than those of any other state estimation method using the same set of sensors reported in the literature.