You might also like...
Check out some other posts from Florida Tech

Call it a matter of pride, national security or a desire for astronomical dominance; there's a sense of urgency within the U.S. government to return to the moon, sparked by China's team of taikonauts, who could land there before American astronauts get back to the lunar surface.
The latest space race is a topic that is making national news. Florida Tech's experts are lending their opinions and insights about the likelihood of a lunar return, and what it might mean.
NASA, with the urging of many politicians, has been racing to get astronauts back to the moon — before the Chinese land taikonauts on the lunar surface. But what’s the rush to return to a place the United States has already been and left 53 years ago? Especially when Mars looms as an enticing option for interplanetary travel. Space experts say there’s plenty of reasons for the urgency: national pride and national security. But also returning to the moon and building habitats would mean long term dominance in space and ensure access to resources that NASA didn’t know where there when the Apollo missions flew. Now with the Chinese making significant progress in human space exploration, the clock is ticking. “The Chinese in the last 20 years have made amazing strides in all aspects of space. They’re sending robots to the moon on a very regular basis. Now they’re doing some pretty amazing activities even on the far side of the moon, and they have a Chinese space station now in Earth orbit,” said Don Platt, associate professor of space systems at Florida Tech. Can China beat NASA to the moon? “The Chinese have really caught up,” said Platt. “I do believe that the Chinese are definitely advancing their efforts on the moon, and are identifying it as a critical aspect of their strategic future in space." When asked about the prospect of Chinese astronauts making it to the moon before NASA's planned Artemis III mission, Platt said he believes it’s a possibility and he cited the efforts China is making to highlight the importance of the nation's space efforts to its own populace. “They have some amazing videos. They’re really engaging the Chinese public, and really using it to do what what we’ve always done in space, and that is to inspire the next generation and to show the world the technical abilities of the Chinese,” said Platt. May 21 - USA Today The race is on, and it's getting a lot of attention. If you're a journalist following this ongoing story, let us help with your coverage.
Dr. Don Platt's work has involved developing, testing and flying different types of avionics, communications and rocket propulsion systems. He also studies astrobiology and biotechnology systems and human deep space exploration tools.
Don is available to speak with media anytime. Simply click on the icon below to arrange an interview today.
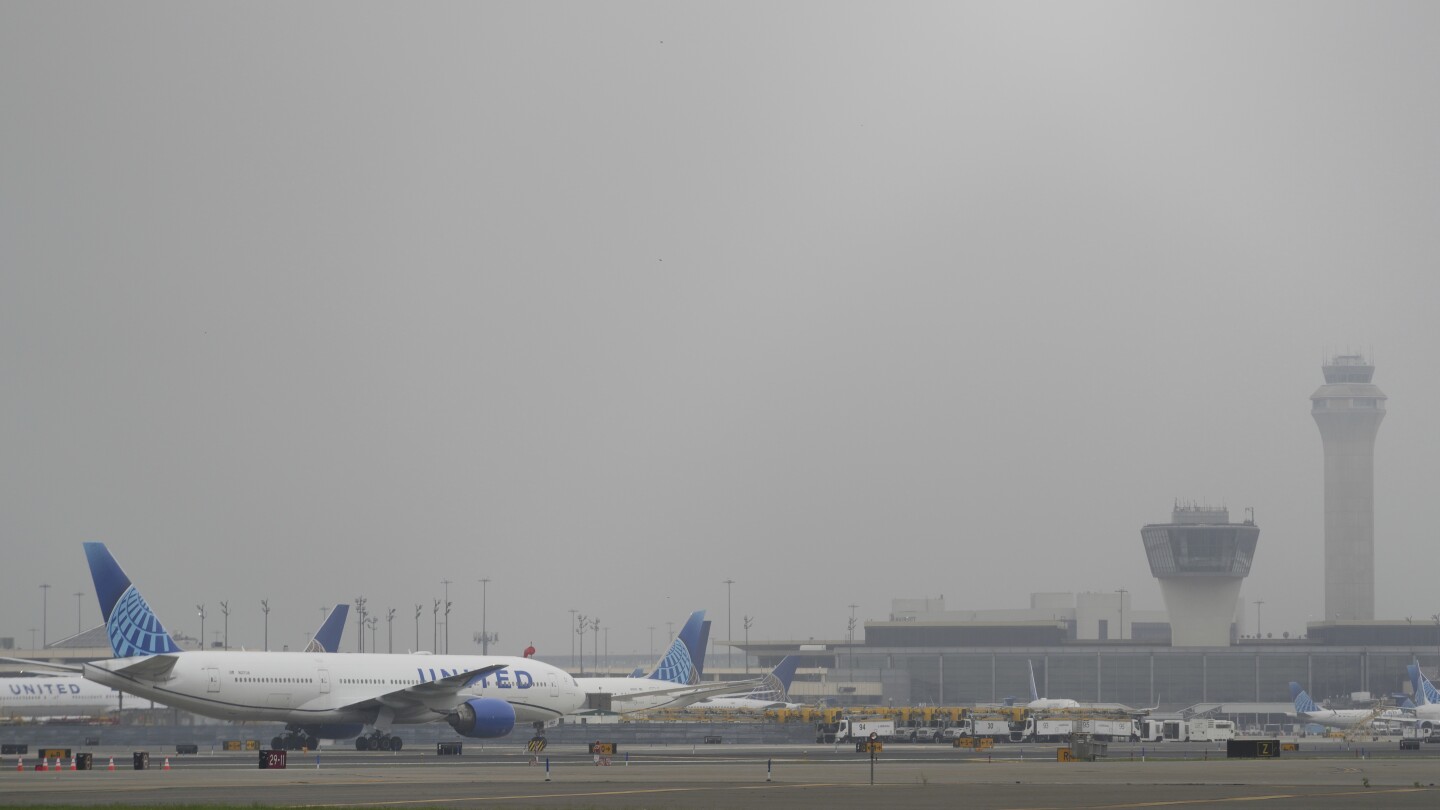
For the second time in two weeks, air traffic controllers directing planes into the Newark, New Jersey, airport briefly lost their radar. The outages have sparked travel chaos, with hundreds of flight delays and cancellations after the FAA slowed air traffic to ensure safety.
The country's aging air traffic control system is in the spotlight. Media, politicians and the public are demanding both solutions for the system and answers on how safe traveling is at the moment. To provide insight, Florida Tech's Margaret Wallace is lending her expert opinion and perspective on the issue.
Margaret Wallace is Assistant Professor of Aviation Management at Florida Institute of Technology, where she teaches Air Traffic Control and Airport Management courses. She spent over 15 years in the industry prior to teaching as an Airport Manager (4 years) at Ramstein Air Base in Germany and an Air Traffic Controller (10+ years) in the U.S. Air Force. “The recent communication failure at Newark Liberty International Airport has raised serious concerns about the safety and dependability of air traffic control systems in the United States. On April 28, 2025, the Newark air traffic facilities lost all radio communication with approximately 20 airplanes for up to 90 seconds due to an equipment breakdown. During the outage, pilots and controllers were unable to communicate. Controllers were unable to maintain aircraft separation during crucial flight phases, and pilots were unable to receive air traffic clearances and instructions. Situations like this, as well as aircraft incidents, bring stress and trauma to the controller's mental state. Most people cannot fathom how much mental stress the controller experiences in everyday job settings. Situations with defective equipment, combined with lengthy work hours due to a scarcity of controllers, appear to have taken their toll based on the fact that several controllers have taken leave for mental stress. This situation posed a safety risk to all planes and passengers. Fortunately, there were no incidents, and everyone remained safe. However, this demonstrated some of the flaws in the outdated air traffic system equipment. Sean Duffy, the new Transportation Secretary, has acknowledged the critical need to improve our current technology. While air travel is generally safe, our current administration must continue to prioritize the upgrade of air traffic systems and increasing the staffing in air traffic facilities. To ensure safety, I believe we should consider having airlines restrict the number of flights available and the Air Route Traffic Command Center to introduce delays to avoid overloading the system.” Margaret Wallace If you're interested in connecting with Margaret Wallace about the ongoing issues at airports across the country, let us help. Contact Adam Lowenstein, Director of Media Communications at Florida Institute of Technology, at adam@fit.edu to arrange an interview today.
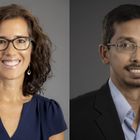
A Florida Tech-led group of researchers was selected to help NASA solve challenges in aviation through its prestigious University Leadership Initiative (ULI) program. Over the next three years, associate professor of computer science and software engineering Siddhartha Bhattacharyya and professor of aviation human factors Meredith Carroll will work to understand the vital role of trust in autonomy.
Their project, “Trustworthy Resilient Autonomous Agents for Safe City Transportation in the Evolving New Decade” (TRANSCEND), aims to establish a common framework for engineers and human operators to determine the trustworthiness of machine-learning-enabled autonomous aviation safety systems.
Autonomous systems are those that can perform independent tasks without requiring human control. The autonomy of these systems is expected to be enhanced with intelligence gained from machine learning. As a result, intelligence-based software is expected to be increasingly used in airplanes and drones. It may also be utilized in airports and to manage air traffic in the future.
Learning-enabled autonomous technology can also act as contingency management when used in safety applications, proactively addressing potential disruptions and unexpected aviation events.
TRANSCEND was one of three projects chosen for the latest ULI awards. The others hail from Embry-Riddle Aeronautical University in Daytona Beach – researching continuously updating, self-diagnostic vehicle health management to enhance the safety and reliability of Advanced Air Mobility vehicles – and University of Colorado Boulder – investigating tools for understanding and leveraging the complex communications environment of collaborative, autonomous airspace systems.
Florida Tech’s team includes nine faculty members from five universities: Penn State; North Carolina A&T State University; University of Florida; Stanford University; Santa Fe College. It also involves the companies Collins Aerospace in Cedar Rapids, Iowa and ResilienX of Syracuse, New York. Carroll and Bhattacharyya will also involve students throughout the project.
Human operators are an essential component of aviation technology – they monitor independent software systems and associated data and intervene when those systems fail. They may include flight crew members, air traffic controllers, maintenance personnel or safety staff monitoring overall system safety.
A challenge in implementing independent software is that engineers and operators have different interpretations of what makes a system “trustworthy,” Carroll and Bhattacharyya explained. Engineers who develop autonomous software measure trustworthiness by the system’s ability to perform as designed. Human operators, however, trust and rely on systems to perform as they expect – they want to feel comfortable relying on a system to make an aeronautical decision in flight, such as how to avoid a traffic conflict or a weather event. Sometimes, that reliance won’t align with design specifications.
Equally important, operators also need to trust that the software will alert them when it needs a human to take over. This may happen if the algorithm driving the software encounters a scenario it wasn’t trained for.
“We are looking at how we can integrate trust from different communities – from human factors, from formal methods, from autonomy, from AI…” Bhattacharyya said. “How do we convey assumptions for trust, from design time to operation, as the intelligent systems are being deployed, so that we can trust them and know when they’re going to fail, especially those that are learning-enabled, meaning they adapt based on machine learning algorithms?” With Bhattacharyya leading the engineering side and Carroll leading the human factors side, the research group will begin bridging the trust gap by integrating theories, principles, methods, measures, visualizations, explainability and practices from different domains – this will build the TRANSCEND framework. Then, they’ll test the framework using a diverse range of tools, flight simulators and intelligent decision-making to demonstrate trustworthiness in practice. This and other data will help them develop a safety case toolkit of guidelines for development processes, recommendations and suggested safety measures for engineers to reference when designing “trustworthy,” learning-enabled autonomous systems.
Ultimately, Bhattacharyya and Carroll hope their toolkit will lay the groundwork for a future learning-enabled autonomous systems certification process.
“The goal is to combine all our research capabilities and pull together a unified story that outputs unified products to the industry,” Carroll said. “We want products for the industry to utilize when implementing learning-enabled autonomy for more effective safety management systems.” The researchers also plan to use this toolkit to teach future engineers about the nuances of trust in the products they develop. Once developed, they will hold outreach events, such as lectures and camps, for STEM-minded students in the community.
If you're interested in connecting with Meredith Carroll or Siddhartha Bhattacharyya - simply click on the expert's profile or contact Adam Lowenstein, Director of Media Communications at Florida Institute of Technology at adam@fit.edu to arrange an interview today.