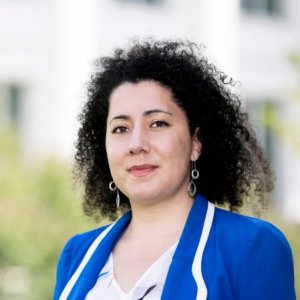
Anna Errore
Assistant Professor in the Practice of Information Systems & Operations Management Emory University, Goizueta Business School
- ATLANTA GA
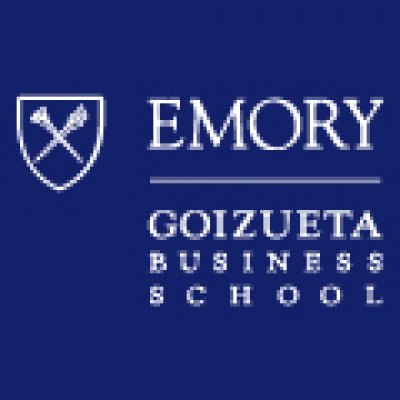
Emory University, Goizueta Business School
View more experts managed by Emory University, Goizueta Business School
Biography
Education
University of Minnesota Carlson School of Management
PhD
Business Administration
2021
minor in Statistics
University of Palermo (Italy)
PhD
Industrial Engineering
2015
University of Palermo (Italy)
MS
Industrial Engineering
2011
University of Palermo (Italy)
BS
Industrial Engineering
2009
Areas of Expertise
Publications
Lifting Statewide Mask Mandates and COVID-19 Cases: A Synthetic Control Study
Medical Care, 60(7), 538-544Petersen, F., Errore, A., & Karaca-Mandic, P.
2022-07-01
Abstract
Importance: As states reopened their economies state and local officials made decisions on policies and restrictions that had an impact on the evolution of the pandemic and the health of the citizens. Some states made the decision to lift mask mandates starting in spring 2021. Data-driven methods help evaluate the appropriateness and consequences of such decisions.
Objective: To investigate the association of lifting the mask mandate with changes in the cumulative coronavirus case rate.
Design: Synthetic control study design on lifting mask mandate in the state of Iowa implemented on February 7, 2021.
Setting: Daily state-level data from the COVID-19 Community Profile Report published by the US Department of Health & Human Services, COVIDcast dataset of the Delphi Research Group, and Google Community Mobility Reports.
Exposures and Outcome: Mask mandate policy lift at the state level. State-day observations of the cumulative case rate measured as the cumulative number of new cases per 100,000 people in the previous 7 days.
Results: The cumulative case rate in Iowa increased by 20%–30% within 3 weeks of lifting the mask mandate as compared with a synthetic control unit. This association appeared to be related to people, in fact, reducing their mask-wearing habits.
Conclusions: Lifting the mask mandate in Iowa was associated with an increase in new COVID-19 cases. Caution should be applied when making this type of policy decision before having achieved a more stable control of the pandemic.
Using definitive screening designs to identify active first-and second-order factor effects
Journal of Quality Technology, 49(3), 244-264Anna Errore, Bradley Jones, William Li, Christopher J Nachtsheim
2017-11-21
Abstract
Definitive screening designs (DSDs) were recently introduced by Jones and Nachtsheim (2011b). The use of three-level factors and the desirable aliasing structure of the DSDs make them potentially suitable for identifying main effects and second-order terms in one stage of experimentation. However, as the number of active effects approaches the number of runs, the performance of standard model-selection routines will inevitably degrade. In this paper, we characterize the ability of DSDs to correctly identify first- and second-order model terms as a function of the level of sparsity, the number of factors in the design, the signal-to-noise ratio, the model type (unrestricted or following strong heredity), the model-selection technique, and the number of augmented runs. We find that minimum-run-size DSDs can be used to identify active terms with high probability as long as the number of effects is less than or equal to about half the number of runs and the signal-to-noise ratios for the active effects are above about 2.0. We also find that if minimum-run-size designs are augmented with four or more runs, the number of model terms that can be identified with high probability increases substantially. Among the model-selection methods investigated, we found that both Lasso and the Gauss–Dantzig selector (both based on AICc) can be used to effectively identify active model terms in the presence of unrestricted models. For models following strong heredity, the SHIM method developed by Choi et al. (2010) was the best among methods tested that were designed for the strong-heredity case.
Benefits and fast construction of efficient two-level foldover designs
Technometrics, 59(1) pp. 48-57Anna Errore, Bradley Jones,William Li &Christopher J. Nachtsheim
2017-01-31
Abstract
Recent work in two-level screening experiments has demonstrated the advantages of using small foldover designs, even when such designs are not orthogonal for the estimation of main effects (MEs). In this article, we provide further support for this argument and develop a fast algorithm for constructing efficient two-level foldover (EFD) designs. We show that these designs have equal or greater efficiency for estimating the ME model versus competitive designs in the literature and that our algorithmic approach allows the fast construction of designs with many more factors and/or runs. Our compromise algorithm allows the practitioner to choose among many designs making a trade-off between efficiency of the main effect estimates and correlation of the two-factor interactions (2FIs). Using our compromise approach, practitioners can decide just how much efficiency they are willing to sacrifice to avoid confounded 2FIs as well as lowering an omnibus measure of correlation among the 2FIs.