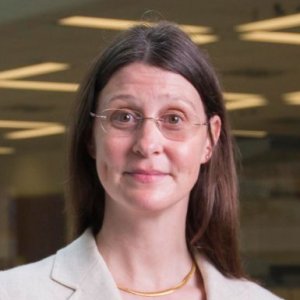
Bridget McInnes, Ph.D.
Associate Professor VCU College of Engineering
- Engineering East Hall, Room E4255, Richmond VA
Dr. McInnes' research is in the area of Natural Language Processing (NLP) with a particular interest in semantics.
Social
Biography
- Word sense disambiguation
- Biomedical text processing
- Semantic similarity and relatedness
- Information extraction
- Literature-based discovery
Industry Expertise
Areas of Expertise
Education
University of Minnesota
Ph.D.
Computer Science
2009
University of Minnesota
M.S.
Computer Science
2004
University of Minnesota
B.S.
Computer Science
2002
Selected Articles
U-path: An undirected path-based measure of semantic similarity
AMIA Annual Symposium Proceedings Archive2014
In this paper, we present the results of a method using undirected paths to determine the degree of semantic similarity between two concepts in a dense taxonomy with multiple inheritance. The overall objective of this work was to explore methods that take advantage of dense multi-hierarchical taxonomies that are more graph-like than tree-like by incorporating the proximity of concepts with respect to each other within the entire is-a hierarchy. Our hypothesis is that the proximity of the concepts regardless of how they are connected is an indicator to the degree of their similarity. We evaluate our method using the Systematized Nomenclature of Medicine Clinical Terms (SNOMED CT), and four reference standards that have been manually tagged by human annotators. The overall results of our experiments show, in SNOMED CT, the location of the concepts with respect to each other does indicate the degree to which they are similar.
Determining the Difficulty of Word Sense Disambiguation
Journal of Biomedical Informatics2014
Automatic processing of biomedical documents is made difficult by the fact that many of the terms they contain are ambiguous. Word Sense Disambiguation (WSD) systems attempt to resolve these ambiguities and identify the correct meaning. However, the published literature on WSD systems for biomedical documents report considerable differences in performance for different terms. The development of WSD systems is often expensive with respect to acquiring the necessary training data. It would therefore be useful to be able to predict in advance which terms WSD systems are likely to perform well or badly on.
This paper explores various methods for estimating the performance of WSD systems on a wide range of ambiguous biomedical terms (including ambiguous words/phrases and abbreviations). The methods include both supervised and unsupervised approaches. The supervised approaches make use of information from labeled training data while the unsupervised ones rely on the UMLS Metathesaurus. The approaches are evaluated by comparing their predictions about how difficult disambiguation will be for ambiguous terms against the output of two WSD systems. We find the supervised methods are the best predictors of WSD difficulty, but are limited by their dependence on labeled training data. The unsupervised methods all perform well in some situations and can be applied more widely.
Evaluating Measures of Semantic Similarity and Relatedness to Disambiguate Terms in Biomedical Text
Journal of Biomedical Informatics2013
In this article, we evaluate a knowledge-based word sense disambiguation method that determines the intended concept associated with an ambiguous word in biomedical text using semantic similarity and relatedness measures. These measures quantify the degree of similarity or relatedness between concepts in the Unified Medical Language System (UMLS). The objective of this work is to develop a method that can disambiguate terms in biomedical text by exploiting similarity and relatedness information extracted from biomedical resources and to evaluate the efficacy of these measure on WSD.
Similarity: Measuring the Relatedness and Similarity of Biomedical Concepts
Association for Computational Linguistics2013
UMLS::Similarity is freely available open source software that allows a user to measure the semantic similarity or relatedness of biomedical terms found in the Unified Medical Language System (UMLS). It is written in Perl and can be used via a command line interface, an API, or a Web interface.
Using PharmGKB to Train Text Mining Approaches for Identifying Potential Gene Targets for Pharmacogenomic Studies.
Journal of Biomedical Informatics2012
The main objective of this study was to investigate the feasibility of using PharmGKB, a pharmacogenomic database, as a source of training data in combination with text of MEDLINE abstracts for a text mining approach to identification of potential gene targets for pathway-driven pharmacogenomics research. We used the manually curated relations between drugs and genes in PharmGKB database to train a support vector machine predictive model and applied this model prospectively to MEDLINE abstracts. The gene targets suggested by this approach were subsequently manually reviewed. Our quantitative analysis showed that a support vector machine classifiers trained on MEDLINE abstracts with single words (unigrams) used as features and PharmGKB relations used for supervision, achieve an overall sensitivity of 85% and specificity of 69%. The subsequent qualitative analysis showed that gene targets “suggested” by the automatic classifier were not anticipated by expert reviewers but were subsequently found to be relevant to the three drugs that were investigated: carbamazepine, lamivudine and zidovudine. Our results show that this approach is not only feasible but may also find new gene targets not identifiable by other methods thus making it a valuable tool for pathway-driven pharmacogenomics research.