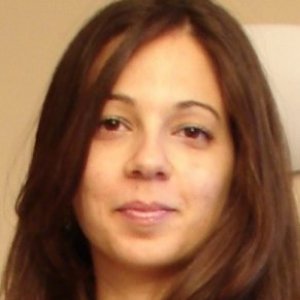
Dr Maria Chli
Reader, Computer Science Aston University
- Birmingham
Dr Chli's work is in the area of artificial intelligence, particularly in applying machine learning techniques.
Social
Biography
Her core research in modelling real-life complex systems and optimising them through coupling multi-agent systems techniques with machine and deep learning, has received multiple awards and has attracted substantial attention from the industry, government, and the media.
For example, her work on trust and reputation in multi-agent interaction settings using Hidden Markov Models is the state of the art in multi-agent trust models.
Similarly, her team's work on automated power brokering resulted in the first reinforcement learning broker agent, AstonTAC.
This success has inspired more current work on deep reinforcement learning architectures for real-time control (e.g., traffic signal control) and transfer learning which have produced outstanding results in their respective areas. Her work on autonomous traffic control has attracted the attention of regional Departments for Transport both in the UK and abroad, which are eager to start trials of our algorithms in the West Midlands area.
This work has also been widely covered by the media (BBC News, Deutsche Well and multiple other outlets).
Dr Chli's £2.4m grant track record includes funding from a diverse set of funders such as Innovate UK, European Commission, The Royal Society, AXA and the Industry.
Areas of Expertise
Accomplishments
Founder
Founder of multi-award-winning CS Industry Club scheme.
REF 2021
Published two 4* and at least eight 3* papers.
Funding
Received £2.4m of research funding. As industrial liaison, doubled department's industrial research funding (£6.3m in six years)
Education
Aston University
Certificate
Learning and Teaching in Higher Education
2007
Imperial College London
PhD
Intelligent Systems
2005
Imperial College London
MEng
Computing
2001
Affiliations
- ALICE Research Group, Systems Analytics Research Institute, SARI.
Media Appearances
Can we make traffic jams a thing of the past?
RNZ radio
2022-06-26
Here's a glimpse of the future, finally, the world's first traffic control system of its kind, they say, using artificial intelligence, designed to make urban traffic jams a thing of the past.
Computer scientist Dr Maria Chli joins Jim from Aston University in Birmingham.
AI traffic light system could make traffic jams a distant memory
EurekAlert! online
2022-05-11
Dr Maria Chli, reader in Computer Science at Aston University, explained: “We have set this up as a traffic control game. The program gets a ‘reward’ when it gets a car through a junction. Every time a car has to wait or there’s a jam, there’s a negative reward. There’s actually no input from us; we simply control the reward system.”
Traffic jams at junctions
BBC online
2022-11-03
Long queues at traffic lights could be a thing of the past, thanks to a new artificial intelligence system developed by Aston University researchers.
Articles
Fully-Autonomous, Vision-based Traffic Signal Control: from Simulation to Reality
ACM2022
Ineffective traffic signal control is one of the major causes of congestion in urban road networks. Dynamically changing traffic conditions and live traffic state estimation are fundamental challenges that limit the ability of the existing signal infrastructure in rendering individualized signal control in real-time. We use deep reinforcement learning (DRL) to address these challenges. Due to economic and safety constraints associated training such agents in the real world, a practical approach is to do so in simulation before deployment. Domain randomisation is an effective technique for bridging the reality gap and ensuring effective transfer of simulation-trained agents to the real world. In this paper, we develop a fully-autonomous, vision-based DRL agent that achieve adaptive signal control in the face of complex, imprecise, and dynamic traffic environments. Our agent uses live visual data (i.e. a stream of real-time RGB footage) from an intersection to extensively perceive and subsequently act upon the traffic environment. Employing domain randomisation, we examine our agent’s generalisation capabilities under varying traffic conditions in both the simulation and the real-world environments. In a diverse validation set independent of training data, our traffic control agent reliably adapted to novel traffic situations and demonstrated a positive transfer to previously unseen real intersections despite being trained entirely in simulation.
Traffic3d: An Open-Source Traffic-Based Interactive Framework to Train AI Agents
SSRN2022
Significant breakthrough in the field of artificial intelligence (AI) has been driven by the use of game environments for training and evaluating autonomous agents. Inspired by the extensive literature on the use of game-based simulation environments, in this paper, we present a novel edition of a 3D-road traffic environment; Traffic3D. Traffic3D is a rich, realistic and extensible simulation platform built to reproduce real-world dynamic and diverse traffic scenarios; incorporating adequate physical behavior and life-like visual appearance of the traffic entities (such as vehicles and transportation infrastructure) as well as environment and road conditions. A seamless interface with python makes it possible to use Traffic3D, not only as a simulator but as a rich training ground for AI-powered autonomous traffic tasks, such as autonomous driving and traffic signal infrastructure optimization. To showcase Traffic3D's capabilities in producing real-world deployable AI agents, we trained an AI agent to achieve fully-autonomous signal control by effectively regulating the traffic flows through the road intersections in {\em real time} based {\em solely} on {\em live} traffic footage. Our empirical analysis demonstrates that our agent while being entirely trained in simulation transfers seamlessly to the real world and reliably adapts to novel traffic situations. To support the research community in the development of new techniques,we have made Traffic3D publicly available at https://traffic3d.org.
Optimal Auction Design for the Gradual Procurement of Strategic Service Provider Agents
arXiv preprint2021
We consider an outsourcing problem where a software agent procures multiple services from providers with uncertain reliabilities to complete a computational task before a strict deadline. The service consumer requires a procurement strategy that achieves the optimal balance between success probability and invocation cost. However, the service providers are self-interested and may misrepresent their private cost information if it benefits them. For such settings, we design a novel procurement auction that provides the consumer with the highest possible revenue, while giving sufficient incentives to providers to tell the truth about their costs. This auction creates a contingent plan for gradual service procurement that suggests recruiting a new provider only when the success probability of the already hired providers drops below a time-dependent threshold. To make this auction incentive compatible, we propose a novel weighted threshold payment scheme which pays the minimum among all truthful mechanisms. Using the weighted payment scheme, we also design a low-complexity near-optimal auction that reduces the computational complexity of the optimal mechanism by 99% with only marginal performance loss (less than 1%). We demonstrate the effectiveness and strength of our proposed auctions through both game theoretical and numerical analysis. The experiment results confirm that the proposed auctions exhibit 59% improvement in performance over the current state-of-the-art, by increasing success probability up to 79% and reducing invocation cost by up to 11%.