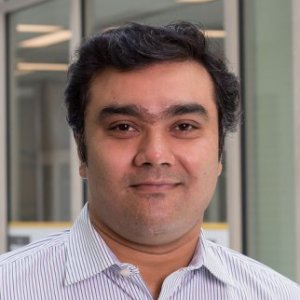
Preetam Ghosh, Ph.D.
Interim Chair and Professor, Department of Computer Science VCU College of Engineering
- Engineering East Hall, Room E4248, Richmond VA
Professor Ghosh researches complex networks and systems biology, using modeling and simulation, applied-AI and network science.
Social
Biography
Industry Expertise
Areas of Expertise
Education
University of Texas at Arlington
Ph.D.
Computer Science and Engineering
2007
University of Texas at Arlington
M.S.
Computer Science and Engineering
2004
Jadavpur University
B.E.
Computer Science and Engineering
2000
Selected Articles
Towards a Unified Pandemic Management Architecture: Survey, Challenges, and Future Directions
ACM Computing SurveysSatyaki Roy, Nirnay Ghosh, Nitish Uplavikar, Preetam Ghosh
2023-09-14
The pandemic caused by Severe Acute Respiratory Syndrome Coronavirus 2 (SARS-CoV-2) has impacted the economy, health, and society. Emerging strains are making pandemic management challenging. There is an urge to collect epidemiological, clinical, and physiological data to make an informed decision on mitigation. Advances in the Internet of Things (IoT) and edge computing provide solutions for pandemic management through data collection and intelligent computation. While existing data-driven architectures operate on specific application domains and attempt to automate decision-making, they do not capture the multifaceted interaction among computational models, communication infrastructure, and data. In this article, we survey the existing approaches for pandemic management, including data repositories and contact-tracing applications. We envision a unified pandemic management architecture that leverages the IoT and edge computing paradigms to automate recommendations on vaccine distribution, dynamic lockdown, mobility scheduling, and pandemic trend prediction. We elucidate the data flow among the layers, namely, cloud, edge, and end device layers. Moreover, we address the privacy implications, threats, regulations, and solutions that may be adapted to optimize the utility of health data with security guarantees. The article ends with a discussion of the limitations of the architecture and research directions to enhance its practicality.
Multimodal Classification: Current Landscape, Taxonomy and Future Directions
ACM Computing SurveysWilliam C. Sleeman, IV, Rishabh Kapoor, Preetam Ghosh
2022-12-15
Multimodal classification research has been gaining popularity with new datasets in domains such as satellite imagery, biometrics, and medicine. Prior research has shown the benefits of combining data from multiple sources compared to traditional unimodal data that has led to the development of many novel multimodal architectures. However, the lack of consistent terminologies and architectural descriptions makes it difficult to compare different solutions. We address these challenges by proposing a new taxonomy for describing multimodal classification models based on trends found in recent publications. Examples of how this taxonomy could be applied to existing models are presented as well as a checklist to aid in the clear and complete presentation of future models. Many of the most difficult aspects of unimodal classification have not yet been fully addressed for multimodal datasets, including big data, class imbalance, and instance-level difficulty. We also provide a discussion of these challenges and future directions of research.
Recent advances on constraint-based models by integrating machine learning
Current Opinion in BiotechnologyPratip Rana, Carter Berry, Preetam Ghosh, Stephen S Fong
2019-12-05
Research that meaningfully integrates constraint-based modeling with machine learning is at its infancy but holds much promise. Here, we consider where machine learning has been implemented within the constraint-based modeling reconstruction framework and highlight the need to develop approaches that can identify meaningful features from large-scale data and connect them to biological mechanisms to establish causality to connect genotype to phenotype. We motivate the construction of iterative integrative schemes where machine learning can fine-tune the input constraints in a constraint-based model or contrarily, constraint-based model simulation results are analyzed by machine learning and reconciled with experimental data. This can iteratively refine a constraint-based model until there is consistency between experimental data, machine learning results, and constraint-based model simulations.
Transcriptional Regulatory Network Topology with Applications to Bio-inspired Networking: A Survey
ACM Computing SurveysSatyaki Roy, Preetam Ghosh, Nirnay Ghosh, Sajal K. Das
2021-10-04
The advent of the edge computing network paradigm places the computational and storage resources away from the data centers and closer to the edge of the network largely comprising the heterogeneous IoT devices collecting huge volumes of data. This paradigm has led to considerable improvement in network latency and bandwidth usage over the traditional cloud-centric paradigm. However, the next generation networks continue to be stymied by their inability to achieve adaptive, energy-efficient, timely data transfer in a dynamic and failure-prone environment—the very optimization challenges that are dealt with by biological networks as a consequence of millions of years of evolution. The transcriptional regulatory network (TRN) is a biological network whose innate topological robustness is a function of its underlying graph topology. In this article, we survey these properties of TRN and the metrics derived therefrom that lend themselves to the design of smart networking protocols and architectures. We then review a body of literature on bio-inspired networking solutions that leverage the stated properties of TRN. Finally, we present a vision for specific aspects of TRNs that may inspire future research directions in the fields of large-scale social and communication networks.