8 min
Expert Insight: NFL Fandom: The Last Cultural Unifier?
In 2024, few cultural touchstones unify America. One of the remaining cultural unifiers is the NFL. It is almost guaranteed that the Super Bowl will be the most watched television program each year. Add Taylor Swift (another rare cultural unifier) attending to watch her boyfriend and an appealing halftime musical guest, and you can have over 120 million people watching the same program at the same time. Nothing else comes close. There is little doubt that the NFL is the undisputed champion of American sports. But how do the various NFL fandoms compare? Which team has the top fandom, and which struggles (struggle is relative here, as the lowest-ranked NFL fandom is still impressive)? This is an interesting question in a couple of ways. First, it reveals something important about the level of connection in different cities. Cities with stronger fan bases tend to have more of a shared identity. Boston residents share more love across their teams (Celtics, Red Sox, Bruins, Patriots) than folks from Tampa Bay. “Sports” cities are fundamentally different. It's also an interesting marketing analysis. Fandoms are people who share passion and love for what are essentially brands. Examining fandom can reveal something critical about how brands that inspire fandom are built. Comparing fan bases can also inflame passions. Sports fans are (often) the ultimate fans as they closely identify with their teams and feel each victory as a personal triumph and each loss as a defeat. Because fans’ identities are tied to their teams, ranking fan bases can feel like an attack. Saying Browns fans aren’t as good of fans as Ravens fans feels like an attack on Cleveland. The deeper perspective motivating this analysis is that fandom is about cultural passion, so what people are fans of largely dictates the tone and content of our societies. A society that loves baseball, country music, and trucks feels very different from one that favors soccer, opera, and Vespas. The fandom rankings are a snapshot in time of how fandom works in the NFL. And remember, the NFL is not just the top sports league in America but also the closest thing we have in 2024 to a shared societal passion. Analyzing Fandoms I have been ranking NFL and other fan bases for more than a decade. These fandom analyses are an example of brand equity analytics, and they use two types of data. The goal is to understand the relationship between market characteristics and fandom outcomes at the league level. We can then evaluate each team based on how it performs relative to league norms. The fandom or market outcome measures include things like data on prices, attendance, and social media following. These are measures of fan engagement. Prices provide a signal of how much market power a team has created. Attendance shows the enthusiasm of fans in the market to pay for tickets and take the time to travel and attend. Social media following reveals how many fans the team has in and out of their home market. Each metric has advantages and limitations. Social media following provides an indication of national fandom, but it also captures casual fans who would never pay for a ticket. The second aspect of the analysis focuses on market potential. NFL markets vary from New York, with a population of 20 million, to Green Bay, with a few hundred thousand. Income levels in San Francisco are far higher than in Jacksonville or Cleveland. I use a range of demographics, but income and population are the major factors. Again, the metrics are good but not perfect. For example, using MSA populations isn’t perfect because teams have different footprints. The Packers are more of a Wisconsin team than a Green Bay team. The teams in New York and LA share a market. Should they each get half of the metro area population? One factor that I do not control for is competition. In the southeast, NFL teams may compete with SEC teams. I have debated this issue (with myself) and have decided to neglect it. This year's analysis includes a significant change from last year. The significant change is that I am not controlling for team performance. Controlling for team performance is helpful because it isolates core or unchanging fandom. This approach has appeal, as we can argue that teams with more passionate fandoms will be more resilient against losing seasons. The downside of controlling for performance is that we get less of a measurement of the fandom's overall value. If a team like Kansas City is on an extended winning streak, then the Chiefs brand is very valuable at the moment. Controlling for winning makes the analysis more about the core, near-permanent passion of a fandom, while not controlling makes the results more relevant to current brand power. The analysis involves three steps. The first step creates measures of each team’s relative fandom outcomes and market potential. The second step develops a statistical model of the relationship between market potential and fandom outcomes. The third step compares each team's fandom outcomes with the statistical model's predictions. The third step is a comparison of actual results versus predicted – the key point is that the prediction is based on leaguewide data. As these analyses are always imperfect, the best way to consider the fandom rankings is as tiers. I like the idea of quadrants. Some brief comments on the members of each quadrant (Elite, Solid, Role Players, Benchwarmers). I will be discussing each fandom on social media. TikTok: @fanalyticspodcast Instagram: @fanalyticsmikelewis YouTube: @fanalyticsmike A bonus figure follow the Quad overviews. The Results Quadrant 1: The Elite The Dallas Cowboys lead the top group of teams, followed by the Packers, Eagles, Chiefs, 49ers, Raiders, Patriots, and Steelers. Sounds a lot like what the man on the street would list as the top NFL brands. The Cowboys and Packers leading the way is no surprise. The Cowboys are second in social following and the leaders in attendance. The Packers are an astonishing fandom story as the team is located in the definitive small market. The Eagles leading the Steelers is going to be troubling in Western Pennsylvania, but the Eagles have more pricing power and more social following. The 49ers are a solid NFL fandom with few weaknesses. The Patriots are in a new era, and it will be fascinating to see if they maintain their top-tier position as Brady and Belichick become memories. The Chiefs' presence in the top group is a change from past years and is due to the shift away from controlling for performance. The Chiefs have a great fandom, but the team’s success currently pumps them up. The Chiefs are in a brand-building phase as the team continues building its dynasty. The question for the Chiefs is where they end up long-term. I don't fully understand the Raiders' ranking. The Raiders are midrange in attendance and social following but do well because are reported to have the highest prices in the league. I suspect this is more an idiosyncrasy of the Las Vegas market than a reflection of significant passionate fandom. Quadrant 2: Solid Performers The Quadrant 2 teams are the Broncos, Giants, Panthers, Seahawks, Saints, Ravens, Texans, and Browns. These are the solid performers of NFL fandoms (brands). These are teams with above expected fandom outcomes for their relative market potentials. The Quadrant 2 clubs are all passionate fanbases (maybe one exception) despite very different histories. For example, the AFC North rival Ravens and Browns differ in both relative history and frequency of winning. Cleveland fandom involves significant character, while the Ravens are a “blue-collar” brand that has been a consistent winner. There are a lot of great stories in Quad 2. The Saints were once the Aints but are now a core part of New Orleans. The Broncos and Giants are great fandoms who are probably angry to be left out of Quad 1. The Panthers' position is unexpected and may be due to some inflated social media numbers. This is the challenge when an analysis is based only on data. When data gets a little weird, like an inflated social media follower count dating back to Cam Newton's days, the results can also get a little weird. This is a teachable moment—do not analyze and interpret data without knowing the context (the data-generating processes). Quadrant 3: Role Players Quadrant 3 fandoms are teams whose fandom outcomes are slightly below average league performance (for similar markets). The Quadrant 3 teams include (in order) the Bills, Falcons, Buccaneers, Jets, Vikings, Bears, Dolphins, and Bengals. There are some interesting teams in Quad 3. The Bills have a great and notorious fandom. Jumping through flaming tables in subzero weather should get you into the top half of the rankings? The big-market Jets and the small-market Bengals have two of the most fascinating QBs in the league. Both clubs could be poised to get to Quad 2 with a Super Bowl or two. Da’Bears may be one of the most disappointing results. A team with an SNL skit devoted to their fandom in a market like Chicago shouldn’t be in Quad 3. Other quick comments: The Falcons need to win a title. Florida is tough for professional teams. The Vikings should play outside. Quadrant 4: Hopium These are the NFL's weakest fandoms, with the key phrase being “the NFL’s.” The Quad 4 teams, in order, are the Lions, Rams, Jaguars, Colts, Titans, Commanders, Chargers, and Cardinals. It’s a lot of teams who have not won regularly and have many moves and name changes. The Lions are poised for a move upward and maybe a sleeping giant of a fandom. They have the most watchable coach in the league and the most surprising celebrity fan. An interesting side story in Quad 4 is the battle for Los Angeles between the Rams (formerly of Saint Louis) and the Chargers (previously San Diego). They play in the same market, but the Rams have won more. But will Herbert lead the Chargers past the Rams? Quad 4 illustrates an important lesson: consistency. The Rams moved from St. Louis and then back to LA. The Chargers went from San Diego to LA. The Colts left Baltimore in the middle of the night. The Titans were the Oilers and moved from Houston to Nashville. The Cardinals were the other NFL team Saint Louis lost. The Commanders should have stopped with their previous name. The Fandom Outcomes / Market Potential Matrix The following figure is a bit of bonus material that may provide some insight into the inner workings of the analysis. The figure below shows the performance of each team on the Fandom Outcome and the Market Potential Indexes. The upper left region features teams with less lucrative markets but above-average fandoms, like the Packers, Steelers, and Chiefs. The lower right region is the teams with below-average fandom outcomes despite high potential markets, like the Commanders, Chargers, and Rams. This pictorial representation is also interesting as it shows teams with similar positions. These similarities can be somewhat surprising. For example, the Lions and Dolphins have very similar profiles despite the differences between Detroit and Miami. Mike Lewis is an expert in the areas of analytics and marketing. This approach makes Professor Lewis a unique expert on fandom as his work addresses the complete process from success on the field to success at the box office and the campaign trail. Michael is available to speak with media - simply click on his icon now to arrange an interview today. Interested in following Future Fandom! Subscribe for free to receive new posts.
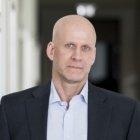