5 min
Why Simultaneous Voting Makes for Good Decisions
How can organizations make robust decisions when time is short, and the stakes are high? It’s a conundrum not unfamiliar to the U.S. Food and Drug Administration. Back in 2021, the FDA found itself under tremendous pressure to decide on the approval of the experimental drug aducanumab, designed to slow the progress of Alzheimer’s disease—a debilitating and incurable condition that ranks among the top 10 causes of death in the United States. Welcomed by the market as a game-changer on its release, aducanumab quickly ran into serious problems. A lack of data on clinical efficacy along with a slew of dangerous side effects meant physicians in their droves were unwilling to prescribe it. Within months of its approval, three FDA advisors resigned in protest, one calling aducanumab, “the worst approval decision that the FDA has made that I can remember.” By the start of 2024, the drug had been pulled by its manufacturers. Of course, with the benefit of hindsight and data from the public’s use of aducanumab, it is easy for us to tell that FDA made the wrong decision then. But is there a better process that would have given FDA the foresight to make the right decision, under limited information? The FDA routinely has to evaluate novel drugs and treatments; medical and pharmaceutical products that can impact the wellbeing of millions of Americans. With stakes this high, the FDA is known to tread carefully: assembling different advisory, review, and funding committees providing diverse knowledge and expertise to assess the evidence and decide whether to approve a new drug, or not. As a federal agency, the FDA is also required to maintain scrupulous records that cover its decisions, and how those decisions are made. The Impact of Voting Mechanisms on Decision Quality Some of this data has been analyzed by Goizueta’s Tian Heong Chan, associate professor of information systems and operation management. Together with Panos Markou of the University of Virginia’s Darden School of Business, Chan scrutinized 17 years’ worth of information, including detailed transcripts from more than 500 FDA advisory committee meetings, to understand the mechanisms and protocols used in FDA decision-making: whether committee members vote to approve products sequentially, with everyone in the room having a say one after another; or if voting happens simultaneously via the push of a button, say, or a show of hands. Chan and Markou also looked at the impact of sequential versus simultaneous voting to see if there were differences in the quality of the decisions each mechanism produced. Their findings are singular. It turns out that when stakeholders vote simultaneously, they make better decisions. Drugs or products approved this way are far less likely to be issued post-market boxed warnings (warnings issued by FDA that call attention to potentially serious health risks associated with the product, that must be displayed on the prescription box itself), and more than two times less likely to be recalled. The FDA changed its voting protocols in 2007, when they switched from sequentially voting around the room, one person after another, to simultaneous voting procedures. And the results are stunning. Tian Heong Chan, Associate Professor of Information Systems & Operation Management “Decisions made by simultaneous voting are more than twice as effective,” says Chan. “After 2007, you see that just 3.4% of all drugs and products approved this way end up being discontinued or recalled. This compares with an 8.6% failure rate for drugs approved by the FDA using more sequential processes—the round robin where individuals had been voting one by one around the room.” Imagine you are told beforehand that you are going to vote on something important by simply raising your hand or pressing a button. In this scenario, you are probably going to want to expend more time and effort in debating all the issues and informing yourself before you decide. Tian Heong Chan “On the other hand, if you know the vote will go around the room, and you will have a chance to hear how others’ speak and explain their decisions, you’re going to be less motivated to exchange and defend your point of view beforehand,” says Chan. In other words, simultaneous decision-making is two times less likely to generate a wrong decision as the sequential approach. Why is this? Chan and Markou believe that these voting mechanisms impact the quality of discussion and debate that undergird decision-making; that the quality of decisions is significantly impacted by how those decisions are made. Quality Discussion Leads to Quality Decisions Parsing the FDA transcripts for content, language, and tonality in both settings, Chan and Markou find evidence to support this. Simultaneous voting or decision-making drives discussions that are characterized by language that is more positive, more authentic, and more even in terms of expressions of authority and hierarchy, says Chan. What’s more, these deliberations and exchanges are deeper and more far-ranging in quality. We find marked differences in the tone of speech and the topics discussed when stakeholders know they will be voting simultaneously. There is less hierarchy in these exchanges, and individuals exhibit greater confidence in sharing their points of view more freely. Tian Heong Chan “We also see more questions being asked, and a broader range of topics and ideas discussed,” says Chan. In this context, decision-makers are also less likely to reach unanimous agreement. Instead, debate is more vigorous and differences of opinion remain more robust. Conversely, sequential voting around the room is typically preceded by shorter discussion in which stakeholders share fewer opinions and ask fewer questions. And this demonstrably impacts the quality of the decisions made, says Chan. Sharing a different perspective to a group requires effort and courage. With sequential voting or decision-making, there seems to be less interest in surfacing diverse perspectives or hidden aspects to complex problems. Tian Heong Chan “So it’s not that individuals are being influenced by what other people say when it comes to voting on the issue—which would be tempting to infer—rather, it’s that sequential voting mechanisms seem to take a bit more effort out of the process.” When decision-makers are told that they will have a chance to vote and to explain their vote, one after another, their incentives to make a prior effort to interrogate each other vigorously, and to work that little bit harder to surface any shortcomings in their own understanding or point of view, or in the data, are relatively weaker, say Chan and Markou. The Takeaway for Organizations Making High-Stakes Decisions Decision-making in different contexts has long been the subject of scholarly scrutiny. Chan and Markou’s research sheds new light on the important role that different mechanisms have in shaping the outcomes of decision-making—and the quality of the decisions that are jointly taken. And this should be on the radar of organizations and institutions charged with making choices that impact swathes of the community, they say. “The FDA has a solid tradition of inviting diversity into its decision-making. But the data shows that harnessing the benefits of diversity is contingent on using the right mechanisms to surface the different expertise you need to be able to see all the dimensions of the issue, and make better informed decisions about it,” says Chan. A good place to start? By a concurrent show of hands. Tian Heong Chan is an associate professor of information systems and operation management. he is available to speak about this topic - click on his con now to arrange an interview today.
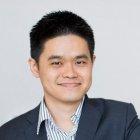