2 min
Aston University researcher takes on leadership role within biomedical engineering
Dr Antonio Fratini is the new chair of the Institute of Mechanical Engineers Biomedical Engineering Division It is one of the largest group of professional biomedical engineers in the UK The specialism merges professional engineering with medical knowledge of the human body, such as artificial limbs and robotic surgery. An Aston University researcher has been given a leading role within the biomedical engineering sector. Dr Antonio Fratini CEng MIMechE has been elected as the new chair of the Biomedical Engineering Division (BmED) of the Institution of Mechanical Engineers (IMechE), one of the largest groups of professional biomedical engineers in the UK. The IMechE has around 115,000 members in 140 countries and has been active since 1847. Biomedical engineering, also known as medical engineering or bioengineering, is the integration of engineering with medical knowledge to help tackle clinical problems and improve healthcare outcomes. Dr Fratini previously served as chair of the Birmingham centre of the division for five years and as vice-chair of the division for one year. His research includes responsible use of AI, 3D segmentation and anatomical modelling to improve surgical training and planning, motor functions and balance rehabilitation. He leads Aston University’s Engineering for Health Research Centre within the College of Engineering and Physical Sciences and has vast experience in the design, development and testing of new medical devices. Currently he is the University’s principal investigator for the West Midlands Health Tech Innovation Accelerator and he has a growing reputation in the UK and internationally within the biomedical engineering profession. He said: “Biomedical engineering is continuously evolving and our graduates will create the future of health tech and med tech for more effective, sustainable, responsible and personalised healthcare. “I am very honoured of this appointment. This three-year post will be a great opportunity to further develop the biomedical engineering profession worldwide and to show Aston University’s commitment to an inclusive, entrepreneurial and transformational impact within the field.” Professor Helen Meese, outgoing chair of the division, said: “I am delighted to see Antonio take on the chair’s position. He has, over the years, contributed significantly to the growth of the Birmingham regional centre and has actively supported me throughout my tenure as chair. I know how passionate he is about our profession and will undoubtedly continue to drive the division forward over the next three years.” Dr Frattini was presented with his new title on 20 June at the IMECHE HQ at 1 Birdcage Walk, London during the Institution’s technology strategy board meeting. For media inquiries in relation to this release, contact Nicola Jones, Press and Communications Manager, on (+44) 7825 342091 or email: n.jones6@aston.ac.uk
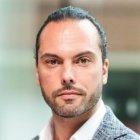