3 min
With Rise in US Autism Rates, Florida Tech Expert Clarifies What We Know About the Disorder
A new report from the Centers for Disease Control and Prevention (CDC) found that an estimated 1 in 31 U.S. children has autism; that's about a 15% increase from a 2020 report, which estimated 1 in 36. The latest numbers come from the CDC’s Autism and Developmental Disabilities Monitoring (ADDM) Network, which tracked diagnoses in 2022 among 8-year-old children. Autism spectrum disorder (ASD) is a neurological disorder that refers to a broad range of conditions affecting social interaction. People with autism may experience challenges with social skills, repetitive behaviors, speech and nonverbal communication. The news has experts like Florida Tech's Kimberly Sloman, Ph.D, weighing in on the matter. She noted that the definition of autism was expanded to include mild cases, which could explain the increase. “Research shows that increased rates are largely due to increased awareness and changes to diagnostic criteria. Much of the increase reflects individuals who have fewer support needs, women and girls and others who may have been misdiagnosed previously," said Sloman. Her insight follows federal health secretary Robert F. Kennedy Jr.'s recent declaration, vowing to conduct further studies to identify environmental factors that could cause the disorder. In his remarks, he also miscategorized autism as a "preventable disease," prompting scrutiny from experts and media attention. “Autism destroys families,” Kennedy said. “More importantly, it destroys our greatest resource, which is our children. These are children who should not be suffering like this.” Kennedy described autism as a “preventable disease,” although researchers and scientists have identified genetic factors that are associated with it. Autism is not considered a disease, but a complex disorder that affects the brain. Cases range widely in severity, with symptoms that can include delays in language, learning, and social or emotional skills. Some autistic traits can go unnoticed well into adulthood. Those who have spent decades researching autism have found no single cause. Besides genetics, scientists have identified various possible factors, including the age of a child’s father, the mother’s weight, and whether she had diabetes or was exposed to certain chemicals. Kennedy said his wide-ranging plan to determine the cause of autism will look at all of those environmental factors, and others. He had previously set a September deadline for determining what causes autism, but said Wednesday that by then, his department will determine at least “some” of the answers. The effort will involve issuing grants to universities and researchers, Kennedy said. He said the researchers will be encouraged to “follow the science, no matter what it says.” April 17 - Associated Press Sloman emphasized that experts are confident that autism has a strong genetic component, meaning there's an element of the disorder that may not be preventable. However, scientists are still working to understand the full scope of the disorder, and much is still unknown. “We know that there’s a strong genetic component for autism, but environmental factors may interact with genetic susceptibility," Sloman said. "This is still not well understood.” Kimberly Sloman’s research interests include best practices for treating individuals with autism spectrum disorder (ASD). She studies the assessment and treatment of problem behavior with methods such as stereotypy, individualized skill assessments and generalization of treatment effects. Are you covering this story or looking to know more about autism and the research behind the disorder? Let us help. Kimberly is available to speak with media about this subject. Simply click on her icon now to arrange an interview today.
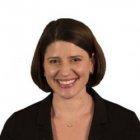