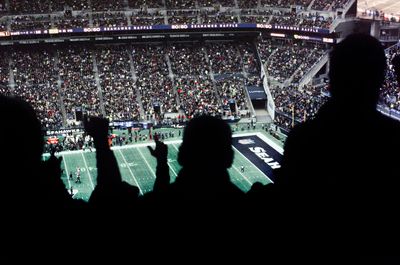
In 2024, few cultural touchstones unify America. One of the remaining cultural unifiers is the NFL. It is almost guaranteed that the Super Bowl will be the most watched television program each year. Add Taylor Swift (another rare cultural unifier) attending to watch her boyfriend and an appealing halftime musical guest, and you can have over 120 million people watching the same program at the same time. Nothing else comes close.
There is little doubt that the NFL is the undisputed champion of American sports. But how do the various NFL fandoms compare? Which team has the top fandom, and which struggles (struggle is relative here, as the lowest-ranked NFL fandom is still impressive)? This is an interesting question in a couple of ways. First, it reveals something important about the level of connection in different cities. Cities with stronger fan bases tend to have more of a shared identity. Boston residents share more love across their teams (Celtics, Red Sox, Bruins, Patriots) than folks from Tampa Bay. “Sports” cities are fundamentally different. It's also an interesting marketing analysis. Fandoms are people who share passion and love for what are essentially brands. Examining fandom can reveal something critical about how brands that inspire fandom are built.
Comparing fan bases can also inflame passions. Sports fans are (often) the ultimate fans as they closely identify with their teams and feel each victory as a personal triumph and each loss as a defeat. Because fans’ identities are tied to their teams, ranking fan bases can feel like an attack. Saying Browns fans aren’t as good of fans as Ravens fans feels like an attack on Cleveland.
The deeper perspective motivating this analysis is that fandom is about cultural passion, so what people are fans of largely dictates the tone and content of our societies. A society that loves baseball, country music, and trucks feels very different from one that favors soccer, opera, and Vespas. The fandom rankings are a snapshot in time of how fandom works in the NFL. And remember, the NFL is not just the top sports league in America but also the closest thing we have in 2024 to a shared societal passion.
Analyzing Fandoms I have been ranking NFL and other fan bases for more than a decade. These fandom analyses are an example of brand equity analytics, and they use two types of data. The goal is to understand the relationship between market characteristics and fandom outcomes at the league level. We can then evaluate each team based on how it performs relative to league norms.
The fandom or market outcome measures include things like data on prices, attendance, and social media following. These are measures of fan engagement. Prices provide a signal of how much market power a team has created. Attendance shows the enthusiasm of fans in the market to pay for tickets and take the time to travel and attend. Social media following reveals how many fans the team has in and out of their home market. Each metric has advantages and limitations. Social media following provides an indication of national fandom, but it also captures casual fans who would never pay for a ticket.
The second aspect of the analysis focuses on market potential. NFL markets vary from New York, with a population of 20 million, to Green Bay, with a few hundred thousand. Income levels in San Francisco are far higher than in Jacksonville or Cleveland. I use a range of demographics, but income and population are the major factors. Again, the metrics are good but not perfect. For example, using MSA populations isn’t perfect because teams have different footprints. The Packers are more of a Wisconsin team than a Green Bay team. The teams in New York and LA share a market. Should they each get half of the metro area population? One factor that I do not control for is competition. In the southeast, NFL teams may compete with SEC teams. I have debated this issue (with myself) and have decided to neglect it.
This year's analysis includes a significant change from last year. The significant change is that I am not controlling for team performance. Controlling for team performance is helpful because it isolates core or unchanging fandom. This approach has appeal, as we can argue that teams with more passionate fandoms will be more resilient against losing seasons. The downside of controlling for performance is that we get less of a measurement of the fandom's overall value. If a team like Kansas City is on an extended winning streak, then the Chiefs brand is very valuable at the moment. Controlling for winning makes the analysis more about the core, near-permanent passion of a fandom, while not controlling makes the results more relevant to current brand power.
The analysis involves three steps. The first step creates measures of each team’s relative fandom outcomes and market potential. The second step develops a statistical model of the relationship between market potential and fandom outcomes. The third step compares each team's fandom outcomes with the statistical model's predictions. The third step is a comparison of actual results versus predicted – the key point is that the prediction is based on leaguewide data. As these analyses are always imperfect, the best way to consider the fandom rankings is as tiers. I like the idea of quadrants.
Some brief comments on the members of each quadrant (Elite, Solid, Role Players, Benchwarmers). I will be discussing each fandom on social media.
TikTok: @fanalyticspodcast Instagram: @fanalyticsmikelewis YouTube: @fanalyticsmike A bonus figure follow the Quad overviews.
The Results Quadrant 1: The Elite The Dallas Cowboys lead the top group of teams, followed by the Packers, Eagles, Chiefs, 49ers, Raiders, Patriots, and Steelers. Sounds a lot like what the man on the street would list as the top NFL brands. The Cowboys and Packers leading the way is no surprise. The Cowboys are second in social following and the leaders in attendance. The Packers are an astonishing fandom story as the team is located in the definitive small market. The Eagles leading the Steelers is going to be troubling in Western Pennsylvania, but the Eagles have more pricing power and more social following. The 49ers are a solid NFL fandom with few weaknesses. The Patriots are in a new era, and it will be fascinating to see if they maintain their top-tier position as Brady and Belichick become memories.
The Chiefs' presence in the top group is a change from past years and is due to the shift away from controlling for performance. The Chiefs have a great fandom, but the team’s success currently pumps them up. The Chiefs are in a brand-building phase as the team continues building its dynasty. The question for the Chiefs is where they end up long-term.
I don't fully understand the Raiders' ranking. The Raiders are midrange in attendance and social following but do well because are reported to have the highest prices in the league. I suspect this is more an idiosyncrasy of the Las Vegas market than a reflection of significant passionate fandom.
Quadrant 2: Solid Performers The Quadrant 2 teams are the Broncos, Giants, Panthers, Seahawks, Saints, Ravens, Texans, and Browns. These are the solid performers of NFL fandoms (brands). These are teams with above expected fandom outcomes for their relative market potentials.
The Quadrant 2 clubs are all passionate fanbases (maybe one exception) despite very different histories. For example, the AFC North rival Ravens and Browns differ in both relative history and frequency of winning. Cleveland fandom involves significant character, while the Ravens are a “blue-collar” brand that has been a consistent winner. There are a lot of great stories in Quad 2. The Saints were once the Aints but are now a core part of New Orleans. The Broncos and Giants are great fandoms who are probably angry to be left out of Quad 1.
The Panthers' position is unexpected and may be due to some inflated social media numbers. This is the challenge when an analysis is based only on data. When data gets a little weird, like an inflated social media follower count dating back to Cam Newton's days, the results can also get a little weird. This is a teachable moment—do not analyze and interpret data without knowing the context (the data-generating processes).
Quadrant 3: Role Players Quadrant 3 fandoms are teams whose fandom outcomes are slightly below average league performance (for similar markets). The Quadrant 3 teams include (in order) the Bills, Falcons, Buccaneers, Jets, Vikings, Bears, Dolphins, and Bengals. There are some interesting teams in Quad 3. The Bills have a great and notorious fandom. Jumping through flaming tables in subzero weather should get you into the top half of the rankings? The big-market Jets and the small-market Bengals have two of the most fascinating QBs in the league. Both clubs could be poised to get to Quad 2 with a Super Bowl or two. Da’Bears may be one of the most disappointing results. A team with an SNL skit devoted to their fandom in a market like Chicago shouldn’t be in Quad 3. Other quick comments: The Falcons need to win a title. Florida is tough for professional teams. The Vikings should play outside.
Quadrant 4: Hopium These are the NFL's weakest fandoms, with the key phrase being “the NFL’s.” The Quad 4 teams, in order, are the Lions, Rams, Jaguars, Colts, Titans, Commanders, Chargers, and Cardinals. It’s a lot of teams who have not won regularly and have many moves and name changes. The Lions are poised for a move upward and maybe a sleeping giant of a fandom. They have the most watchable coach in the league and the most surprising celebrity fan. An interesting side story in Quad 4 is the battle for Los Angeles between the Rams (formerly of Saint Louis) and the Chargers (previously San Diego). They play in the same market, but the Rams have won more. But will Herbert lead the Chargers past the Rams?
Quad 4 illustrates an important lesson: consistency. The Rams moved from St. Louis and then back to LA. The Chargers went from San Diego to LA. The Colts left Baltimore in the middle of the night. The Titans were the Oilers and moved from Houston to Nashville. The Cardinals were the other NFL team Saint Louis lost. The Commanders should have stopped with their previous name.
The Fandom Outcomes / Market Potential Matrix The following figure is a bit of bonus material that may provide some insight into the inner workings of the analysis.
The figure below shows the performance of each team on the Fandom Outcome and the Market Potential Indexes. The upper left region features teams with less lucrative markets but above-average fandoms, like the Packers, Steelers, and Chiefs. The lower right region is the teams with below-average fandom outcomes despite high potential markets, like the Commanders, Chargers, and Rams. This pictorial representation is also interesting as it shows teams with similar positions. These similarities can be somewhat surprising. For example, the Lions and Dolphins have very similar profiles despite the differences between Detroit and Miami.
Mike Lewis is an expert in the areas of analytics and marketing. This approach makes Professor Lewis a unique expert on fandom as his work addresses the complete process from success on the field to success at the box office and the campaign trail.
Michael is available to speak with media - simply click on his icon now to arrange an interview today.
Interested in following Future Fandom! Subscribe for free to receive new posts.
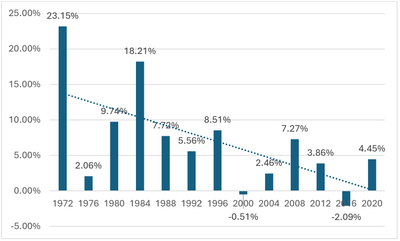
The 2024 Presidential campaign has been a roller coaster ride this summer. The upheavals are so fast and unprecedented that the reaction to each event often seems too muted. An assassination attempt and sudden pre-convention withdrawal? In a past generation, these events would be decisive, but in 2024, they seem like just the latest blip in the news cycle. The polls never seem to move more than a couple of points. In such an oddly volatile but also stable environment, our best bet to understanding what is going to transpire during the last 100 days of the election cycle is to look at data that gets to the heart of how voters view the candidates. My choice of fundamental data or essential metric is candidate fandom.
Fandom is an unusual metric in politics, but it should be more common. Fandom is about passion for and loyalty to a cultural entity, be it a team, singer, university, or even politician. In fact, MAGA Trump supporters and Bernie Bros share many characteristics with Swifties and Lakers fans. Fans of all these things show up, spend, wear branded apparel, and fiercely defend the object of their fandom. The politicians who inspire fandom, such as AOC, Donald Trump, Barack Obama, and Marjorie Taylor Green, enjoy many advantages and are the celebrities of the political world.
Fandom is critical in politics because fans are loyal, engaged, and resilient. Fans are not casual potential voters who may change preferences and are unlikely to make an effort to stand in line to vote. Fans are the voters who will show up rain or shine and who can’t be swayed. In 2024, a fan will interpret a conviction of their candidate as political “lawfare” rather than evidence of criminality. Also, in 2024, a fan will make excuses for signs of aging that would result in children taking a senior’s car keys.
The flip side of fandom, anti-fandom, is also a powerful political force. Indeed, politics may be the cultural context in which anti-fandom has the most impact. Taylor Swift may have haters, but these anti-Swifties are not buying tickets to see Katy Perry in protest. But in politics, hatred of a candidate might be as powerful a tool for generating a vote as fandom. Joe Biden’s 2020 campaign was notoriously bad at drawing crowds, suggesting he inspired little passion. In contrast, Trump’s rallies looked like rabid sports crowds complete with matching hats. However, the hatred and fear of Donald Trump inspired sufficient anti-fandom to make Biden competitive.
Of course, fandom doesn’t entirely decide elections. In most elections, there isn’t all that much fandom or passion. Beyond the presidency and senatorial contests, most candidates are barely known, and identity factors (race, gender, party affiliation) and candidate awareness are the determining factors. Even in presidential elections, get-out-the-vote efforts (ballot harvesting) and election regulations (voter suppression) combined with effective marketing to the few percent of swing (low information) voters are often the determining factors.
Looking toward the future, fandom may be an increasingly salient political metric for multiple reasons. First, the last two decades have witnessed many candidates raised quickly from obscurity with somehow Hollywood-worthy origin stories (Barack Obama, AOC, JD Vance, etc.). In the modern media environment, candidates’ reputations (brands) are increasingly the product of marketing narratives rather than a lifetime of real-world accomplishments. In this new world of politics, fandom will be a critical metric.
Second, with the increasing diversity of the American electorate, voting will be increasingly based on identity rather than ideology. Identity-based voting segments are likely to be driven by fandom (and anti-fandom) rather than policy. We see a form of this in 2024, as high inflation has barely made a dent in voters’ preferences for the two parties. A fragmented electorate comprised of racial and gender segments whose preferences are driven by fandom and anti-fandom will lead to increasingly negative campaigns featuring ads highlighting the threat of the non-preferred party’s candidates. When voters are focused on identity, negative advertising becomes the ideal method to use fear to create anti-fandom (hate) to motivate turnout.
Kamala Harris versus Donald Trump Barring further disruptions, the matchup is set for the 2024 presidential contest (as of this writing, we do not know the Democratic VP). We do know the matchup between Donald Trump and Kamala Harris is a contest between polarizing figures. Donald Trump is a movement candidate who has redefined the Republican party. He inspires passionate fandom from his followers and amazing antipathy from major media and cultural outlets. Harris is also polarizing. In the immediate aftermath of Biden’s withdrawal, Harris received massive media and donor support. However, Harris has not demonstrated any significant national voter appeal, and her time as VP has generated ample blooper real material.
My approach to assessing the race is to examine each candidate's fandom and anti-fandom. Fandom is the candidate’s core, resilient support, while anti-fandom is about antipathy. Fandom and anti-fandom are especially powerful metrics for a candidate because they are relatively fixed after a candidate gains high awareness. Once an individual identifies with the candidate (e.g., they are on the same team), an attack on the candidate is an attack on the individual. This means attack ads do not work because fans feel they are being attacked. Anti-fans are also important because they constrain a candidate’s support. A Trump anti-fan is unpersuadable by efforts from the Trump campaign because their identity is steeped in opposition to him. Fans and anti-fans are trapped in a cycle of confirmation bias where all information is processed to fit their fandom.
I use data from the Next Generation Fandom Survey to assess candidate fandom and anti-fandom. The Next Generation Fandom Survey involves a nationwide sample of the U.S. population regarding fandom for sports and other cultural entities. In the 2024 edition, political figures such as Donald Trump, Joe Biden, Kamala Harris, and RFK Jr were included. The survey captured responses from 2053 subjects split evenly across the four primary generations (Gen Z, Millennials, Gen X, and Baby Boomers), and the sample is representative in terms of racial background. The survey does not focus on likely or registered voters, so the results reflect overall societal sentiments rather than the electorate's opinions. The critical survey question asks subjects to rate how much of a fan they are of a celebrity on a 1 to 7 scale. In the following discussion, individuals who rated their fandom a 6 or 7 on the 7-point scale are categorized as Fans, while those who rate their fandom a 1 or 2 are classified as Anti-Fans.
Table 1 shows the Fandom and Anti-Fandom rates for the entire sample. Donald Trump has a 27% fandom rate compared to Harris's 21%. The fandom rate is crucial because it identifies the candidate's core support. It also indicates something important about the candidate’s potential likability. In terms of anti-fandom, Harris has a slightly higher Anti-Fandom rate. Anti-Fandom is also critical as it shows the percentage of people who hate a candidate. The data suggests that Americans find Harris to be more dislikable than Trump. Notably, the anti-fandom rates are significantly higher than the fandom rates. The American public has significant disdain for politicians. The high anti-fandom rates are both the product of past negative advertising and the cause of future negative campaign strategies.
Table 1: Candidate Fandom and Anti-Fandom Table 2 reports fandom rates based of the two gender segments. Trump has a 7%-point advantage with men and a surprising 4% advantage with women. This is a stunning result as Trump is generally regarded as having weakness with female voters. However, this weakness shows up in the anti-fandom rates. In the male segment, Trump has a 5%-point advantage in anti-fandom (fewer anti-fans), but a 3% disadvantage in the female segment. This reveals that Trump is polarizing to women, and almost half of women find Trump to be highly dislikable. This finding is why the Harris campaign is likely to use advertising that casts Trump as misogynistic or a threat to women to motivate turnout by female voters.
Table 2: Candidate Fandom by Gender Table 3 shows the fandom rates for the two younger demographic segments: Gen Z and Millennials. This Table also shows Trump’s relative performance versus Biden (in parentheses in the last column). Trump enjoys higher fandom and lower anti-fandom than Harris in both the Gen Z and Millennial segments. In terms of fandom, Trump is plus 6% in Gen Z and plus 11% with Millennials. Critically, Harris outperforms Biden. The Gen Z anti-fandom gap between Trump and Biden favored Trump by 6% points. However, this gap shrinks to just 1% point when Harris is the comparison. The data suggests that Harris is stronger with Gen Z than Biden.
Table 3: Candidate Fandom in Younger Generations Table 4 reports the fandom rates based on a racial segmentation scheme. Specifically, the sample is divided into White and Non-White categories. This is a crude segmentation, but it illustrates some essential points. Trump enjoys a significant 14% positive fandom advantage in the White demographic. He also enjoys a 10-point edge in (lower) anti-fandom. The pattern essentially reverses in the Non-White segment, as Harris has a 10-point advantage in fandom and a 17-point edge in anti-fandom. Trump’s anti-fandom in the Non-White segment is critical to the campaign. Nearly half of this segment has antipathy or hate for Trump. This high anti-fandom suggests an opportunity for the Harris campaign to emphasize racial angles in their attacks on Trump.
Table 4: Candidate Fandom by Race In addition to fandom and anti-fandom rates across demographic categories, insights can be gleaned by looking at segmentation variables that reflect cultural values or personality. Table 5 shows fandom and anti-fandom rates for Trump and Harris for segments defined by fandom for Taylor Swift (Swifties) and Baseball.
The Swifties skew towards Harris. The implication is that young women engaged in popular culture have more positive fandom for Harris and more negativity toward Trump. This is unsurprising given the content of the popular culture and Swift’s personal liberalism. The Swiftie segment shows a much stronger skew for Harris than all but the Non-White segment. Examining the data at a cultural level is vital as it indicates that it isn’t necessarily youth or gender where Harris has an advantage but a combination of youth, gender, and a specific type of cultural engagement.
The table also includes fandom rates for baseball fans. In the Baseball Fan segment, Trump enjoys an 8% point fandom advantage and a 7% anti-fandom advantage (lower anti-fandom). Like the case of the Swifties, the fandom rates of Baseball Fans reveal something about Trump’s core support. Baseball is a very traditional game with an older fan base, and traditionalism is probably the core value of Trump fans. Trump’s negative advertising is likely to focus on the threats to traditional values (i.e., Harris is a San Francisco liberal).
Table 5: Candidate Fandom and Cultural Segments Commentary and Prediction Fandom is a powerful metric for predicting political success, but like most data points, it doesn’t tell the whole story. Fandom is a measure of unwavering core support while anti-fandom measures the group that will never support and is likely to show up to vote against a candidate. Examining fandom rates across multiple segments reveals that Harris’ core support is concentrated in specific cultural and racial segments. The analysis also suggests that Trump's core support is broader than is usually acknowledged and that his main problem is significant anti-fandom with women and minorities. Harris’ problem is a lack of love, while Trump’s is too much hate.
Notably, I am not paying too much attention to the current wave of excitement and enthusiasm surrounding Harris. The recent enthusiasm is likely more a manifestation of the Democratic base’s hopes and a relentless media onslaught than an actual increase in passion for Harris. Maybe there will be a permanent shift upward in Harris’s fandom, but I don’t see any logic for why this would occur. Harris isn’t suddenly more likable or aspirational than she was last month. The argument that the American people are becoming more acquainted with her is dubious, given that she has been the Vice President or a major presidential candidate for almost five years.
What are the implications for the upcoming election? Voting is not only about fandom or hate, so we must consider some additional factors. For instance, many potential voters lack passion and knowledge and are more prone to vote based on identity rather than ideology. If a region or demographic segment consistently votes for a party 75% of the time, that’s voting more based on fixed identities than current societal conditions. The American electorate has many of these types of fixed-preference voter segments. Furthermore, as the American electorate becomes more diverse, identity-based voting seems to be making presidential contests more predictable. The baseline seems to be that the Democratic candidate will win the popular vote by a few percentage points, and the Electoral College will come down to a few states, such as Michigan, Pennsylvania, and Wisconsin. Examining past electoral maps shows far more shifting of states across elections. Now, all but a handful of states are regarded as non-competitive.
The Figure below shows the presidential popular vote margins for the last 50 years. It shows a trend towards smaller margins for the winning candidate, which is at least partly due to growing ethnic diversity and more fixed (at least in the near and medium terms) identity-based voting. Over the last 13 cycles, the margin of victory has dropped by about 1% every four years. Demographic change has also locked in a high baseline level of support for Democratic candidates. The last time a Republican won the popular vote was in 2004, with George Bush as the incumbent.
Figure 1: Presidential Vote Margin 1972 to 2020 In addition to shrinking election margins, demographic change promises to change future campaign tones. The increasing relevance of fandom and anti-fandom, combined with the growing diversity of the electorate, will make 2024 an extremely negative campaign.
The 2024 election will be determined by identity-based demographic trends and negative (anti-fandom) marketing campaigns. Demographics are destiny, and America is changing rapidly in ways that make it increasingly difficult for the Republicans to win the popular vote. It doesn’t matter if the Democrat is Harris, Newsom, Clinton, or Whitmer while the Republican is Rubio, Haley, Cruz, or Burgum. The baseline is probably 52% to 48%, D to R. Candidate fandom and anti-fandom probably shift the vote 2 or 3 percent in either direction.
The correlation of demographic traits with voting behaviors creates incentives for campaign strategies that focus on identity. Republicans are eager to shift some percentage of Black or Hispanic voters to their cause because it simultaneously reduces the Democrats' base and grows Republican totals. In contrast, Democrats need to motivate marginal voters in the female, Black, and Hispanic segments to turn out. Fear-based appeals are the most effective tool for both parties' goals.
Negative messaging is also prevalent because of the general view of politicians. Politicians tend to inspire more antipathy (anti-fandom) than admiration (fandom). The fandom data shows this, as both candidates have far more anti-fans than fans (this holds with other politicians) . The modern election calculus is, therefore, focused on aggressive negative ads that inspire marginal voters to take the initiative to vote against a hated candidate. Passion drives behavior, and it's far easier to drive fear and hatred of a candidate than to inspire passion and admiration.
Considering the fandom data and the current electorate, I have two predictions. First, we will witness an incredibly nasty race. Harris’s best bet is to demonize Trump to motivate the anti-Trump voters to turn out. The American culture of 2024 includes constant repetition that many Democratic voting constituencies are marginalized and threatened. These segments are best motivated by using messages that cast the Republicans as the danger or oppressor. Women will fear losing reproductive rights, and African Americans will be primed with threats to voting rights.
Trump will also employ negative messaging, but Trump’s adoption of a negative campaign comes from a slightly different motivation. Trump’s core support consists of conservatives who are frustrated by a lack of cultural power and representation. This group is looking for someone who will fight for their values. This desire for a “fighting advocate” explains much of Trump’s appeal, as his supporters are enthusiastic about his “mean tweets and nicknames.” There will also be fear-based advertising as Harris will be positioned as wanting to defund police and open the border.
Second, Trump wins in a close contest. Comparing Trump’s and Harris’ fandom and anti-fandom suggests the Harris campaign faces an uphill challenge. Despite the current blitz of enthusiasm for Harris as a replacement for a failing Joe Biden, her “brand” has not shown an ability to stimulate passion, and her dislike levels exceed Trump's. It seems unlikely that she will be able to inspire fans. While Trump has a significant fanbase and weaknesses in terms of strong anti-fandom levels in minority and cultural segments, he probably beat Clinton in 2016 because her anti-fandom was equivalent to his. In contrast, he lost to Biden because Biden had less anti-fandom (in 2020). Kamala Harris seems more like Clinton than Biden, so look for a similar outcome as in 2016.
The bottom-line prediction: An exceptionally negative campaign, with Trump’s greater baseline fandom and Harris’s charisma deficit leading to a narrow Trump victory. As in 2016,Trump wins the Electoral College while losing the popular vote.
Addendum: Future Fandom Lesson The structure of the American electorate and the propensity of people to vote based on identity rather than ideology mean that negative campaigns are the standard in the near future. The essential observation is that demographic trends create an electorate that is more a collection of identity segments than a homogeneous population that varies in ideology. An increasingly diverse electorate likely means increasingly negative presidential campaigns as negative or fear-based appeals are especially effective when elections focus on threats to identity groups. The tragedy of this situation is that the negative messages of campaigns amplify racial division and acrimony. When the next election occurs, the electorate is even more polarized, and negative or fear-based appeals are again the most effective.
Mike Lewis is an expert in the areas of analytics and marketing. This approach makes Professor Lewis a unique expert on fandom as his work addresses the complete process from success on the field to success at the box office and the campaign trail.
Michael is available to speak with media - simply click on his icon now to arrange an interview today.
Interested in following Future Fandom! Subscribe for free to receive new posts.